Facility location in hierarchical systems poses a multifaceted challenge, impacting cost optimization, service level enhancement, and the overall reliability of critical services like healthcare, emergency response, and telecommunications. This blog explores how Generative AI, in combination with Mathematical Optimization, can offer a transformative approach to addressing the complexities of facility location within hierarchical systems.
Understanding the Complexity of Hierarchical Systems and Challenges in Facility Location
Hierarchical systems refer to complex organizational structures or systems where various components or entities are organized into multiple levels or layers, each serving a specific function or role within the larger system. These levels interact with one another, and often there is a flow of materials, information, or services between these levels. Here are some examples of hierarchical systems:
• Healthcare System: Healthcare systems often have a hierarchical structure with different levels of care facilities. For example, in a three-level healthcare system, patients typically start at local clinics or primary care facilities for routine check-ups and minor health issues. If a patient’s condition requires more specialized care or advanced diagnostics, they may be referred to a hospital, which represents a higher level in the hierarchy. In cases of extremely complex or critical conditions, patients might be transferred to regional hospitals, which are at the top of the hierarchy, offering specialized treatments and surgeries.
• Production-Distribution System: In manufacturing and distribution networks, hierarchical systems are common. Manufacturers have multiple layers of facilities, including production plants, distribution centers, and retail outlets. Raw materials flow from suppliers to production facilities, where they are transformed into finished products. These products then move through distribution centers before reaching end consumers through retail outlets or e-commerce channels. The coordination of these facilities is essential for efficient production and delivery.
• Emergency Medical Services (EMS): EMS systems often operate within hierarchical structures. In emergency situations, patients may first receive care from local paramedics or EMTs who provide initial medical attention at the scene. Depending on the severity of the medical issue, patients might then be transported to higher-level facilities, such as regional trauma centers or specialized hospitals, for more advanced treatment. This hierarchical approach ensures that patients receive appropriate care based on the complexity of their conditions.
• Telecommunications System: Telecommunications networks are hierarchical in nature. They consist of various components, including cell towers, switching stations, data centers, and user devices. At the lowest level are cell towers that provide coverage to specific geographic areas. These towers are connected to regional switching stations, which, in turn, connect to larger data centers. The hierarchical design ensures efficient data routing and communication across vast networks.
• Education System: Educational systems often have a hierarchical structure with various levels of institutions. For example, students start at the primary or elementary school level and then progress to secondary or high schools. Beyond that, there are institutions of higher education such as colleges and universities. Within universities, there are further hierarchies, including faculties and departments. This hierarchical structure allows students to advance through their education while gaining more specialized knowledge at each level.
These examples demonstrate that hierarchical systems are prevalent in various domains and are characterized by different levels or echelons. The interactions and material flows between these levels play a crucial role in the functioning and efficiency of the overall system.
The selection of optimal facility locations within hierarchical systems is a crucial endeavor, given its profound effects on service levels, operational costs, and system reliability. Facility failures during disruptions, whether caused by natural disasters or unforeseen crises, can lead to service interruptions and increased costs. The manual determination of optimal locations in such intricate systems, marked by complex material flows between facilities, presents significant challenges.
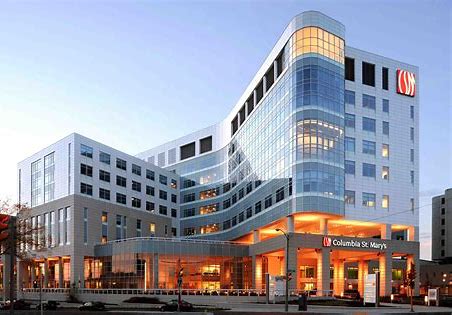
The Power of Mathematical Optimization
Mathematical Optimization, particularly Mathematical Programming or decision optimization, has been instrumental in tackling facility location problems. This approach empowers decision-makers to discern the most favorable decisions from an expansive range of choices, aligning with predefined criteria like profitability, service level maximization, and resource utilization efficiency. Optimization models accurately capture the essential aspects of the business problem, undergo validation against historical data, and are then solved using potent optimization solvers.
Hierarchical Facility Location Problem (HFLP) models represent a specific category of optimization models tailored for hierarchical systems. These models aim to identify optimal facility locations that align with one or more objectives, such as cost minimization and service coverage maximization. Notably, the Hierarchical Maximal Covering Location Problem (HMCLP) focuses on maximizing the number of customer demands covered by facilities, exemplifying one key objective within HFLP.
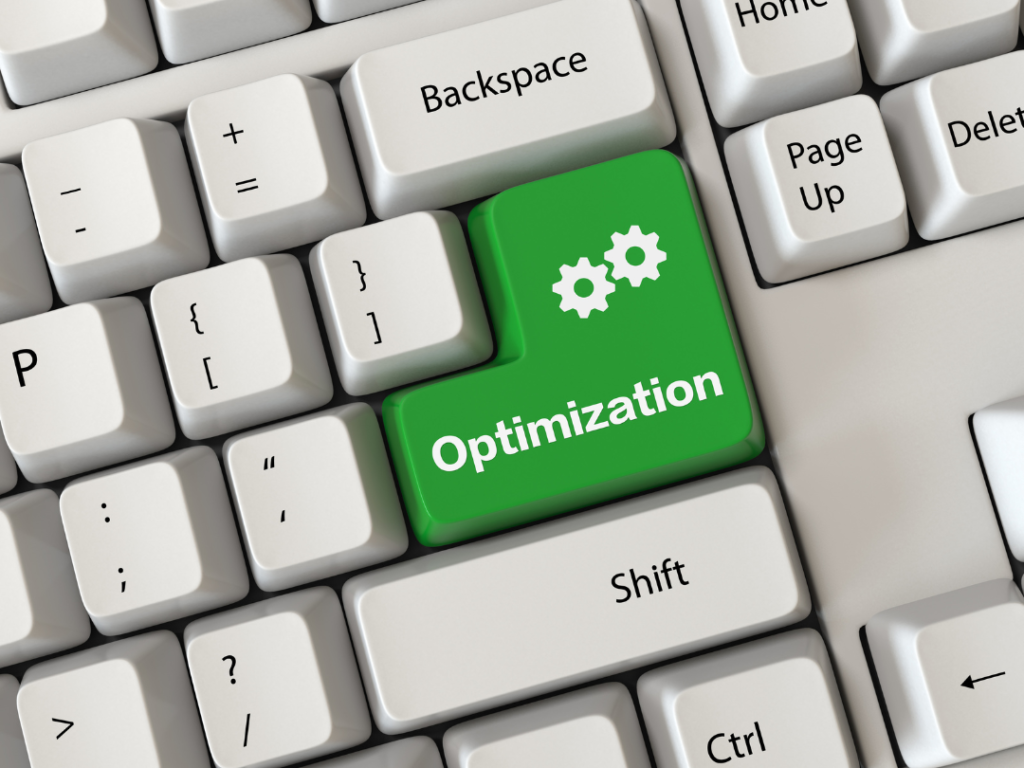
The Role of Generative AI
Generative AI, a subset of artificial intelligence, introduces a new dimension to solving complex problems such as facility location within hierarchical systems. Here’s a closer look at how Generative AI works and how it can be integrated with Mathematical Optimization:
1. Creative Solution Generation: Generative AI employs advanced techniques, including neural networks and deep learning, along with large datasets to generate innovative and imaginative solutions. In the context of facility location, Generative AI can explore a broader range of potential facility locations and configurations than traditional methods.
2. Exploration of Unconventional Options: Unlike traditional methods constrained by pre-existing assumptions, Generative AI is not limited by conventional thinking. It can propose unconventional facility locations that human decision-makers may not have considered, potentially leading to breakthrough solutions.
3. Data-Driven Insights: Generative AI relies on data-driven insights to make informed suggestions. It can analyze historical data, real-time information, and other relevant factors to generate facility location scenarios grounded in data.
4. Scenario Diversification: Generative AI can rapidly create and evaluate a multitude of facility location scenarios. This diversification of options enables decision-makers to explore a broader spectrum of possibilities, including those that might not have been apparent through traditional methods.
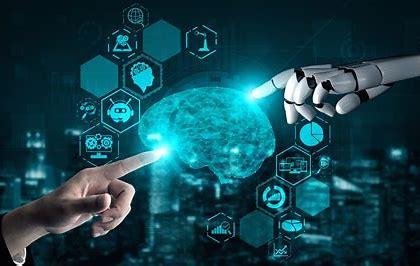
Combining Generative AI and Mathematical Optimization
The integration of Generative AI and Mathematical Optimization offers a powerful synergy that empowers decision-makers to make more informed, data-driven choices within facility location problems for hierarchical systems:
1. Scenario Generation: Generative AI can generate a wide array of imaginative and unconventional facility location scenarios, significantly expanding the decision space.
2. Objective Evaluation: Mathematical Optimization, known for its precision in modeling, can evaluate these scenarios against various objectives, such as cost minimization, service coverage maximization, and reliability enhancement.
3. Real-time Adaptation: In rapidly changing environments or during disruptions, Generative AI can continuously generate and refine facility location scenarios. Mathematical Optimization provides real-time evaluation and adaptation, ensuring that facility locations remain aligned with evolving objectives and constraints.
4. Optimal Decision Making: By combining the creative power of Generative AI with the analytical rigor of Mathematical Optimization, decision-makers can identify optimal facility locations that not only meet immediate goals but also consider long-term sustainability and adaptability.
Conclusion
Facility location problems within hierarchical systems are intricate puzzles with far-reaching implications. Mathematical Optimization has been an instrumental tool in addressing these challenges, but the integration of Generative AI heralds a new era of possibilities. By harnessing the creative power of Generative AI and the precision of Mathematical Optimization, decision-makers can unlock innovative solutions that ensure the profitability and reliability of their hierarchical systems in an ever-changing world. The amalgamation of these technologies promises to reshape the landscape of facility location in hierarchical systems, paving the way for smarter, more resilient infrastructure and services.