In every business, there is some type of forecasting occurring. For example, Walmart recently ran demand prediction for more than 100,000 different products over 4,700 stores in the United States. Customer service centers may predict the demand of call volume every day or to optimize the scheduling of agents. Forecasting can help retail companies solve over-stocking and short-of-supply problems and optimize inventory planning. What is more, data-driven techniques can help companies better understand the effectiveness of their business strategy and improve their marketing response. With the application of data science techniques, sound predictions of demand and trends are no longer luxury items. Not only can millions of dollars be saved, but customer satisfaction can be greatly improved.
Keys to make your demand forecasting model successful include:
- Understand your data – is machine learning always better?
- Understand your business – feature engineering
- Empowering demand forecasting with SPSS + Planning Analytics
1. Understand your data - is machine learning always better?
“Garbage in – garbage out” is a recognition of poor quality which leads to unreliable data output. The quality of the data is mostly determined by 3 factors: The amount of data, data granularity, and randomness.
These 3 factors usually bond with each other. The amount of data is the base of analytics. As for modeling, lack of records (rows) might bring a high variance. Data granularity determines the lowest-level information data scientists could dig out. The lower the granularity, the harder prediction will be. In real business, data granularity problems would come in two dimensions; one is from geography or stage of the supply chain, the other is the dat e(year-month-week-day) with tim e(hour-minute-second). For example, under the scenario of a franchise business with 10 warehouse centers and 1,000 retails centers across the country, even for the same product, techniques for predicting company level, warehouse level, and retail center level will be completely different. And with the change of data granularity, some features may become unavailable, such that monthly promotion predictions may not work if they care about quarterly sales.
Randomness is always the key issue. During COVID-19, most of the demand forecasting models are facing great challenges brought by the uncertainty of the future, especially machine learning models. Complicated neural networks are not necessarily a guarantee to better performance, sometimes it may be worse than a simple moving average. How to avoid things like this happening? It should always start from evaluating the nature of data and letting the data itself tell the story.
2. Understand your business - feature engineering
- Marketing calendars
- Product attributes
- Store location attribute
- Lag features from past
- External data: holidays, weather, etc.
3. Empowering demand forecasting with SPSS + Planning Analytics
SPSS Modeler is a data mining tool to build predictive models and conduct other analytics tasks. It has a visual interface that allows users to leverage statistical and machine learning algorithms with ‘drag and drop’ rather than programming.
Planning Analytics (Formerly Cognos TM1) is a business performance management software suite designed to implement collaborative planning, budgeting, and forecasting solutions, interactive ‘what-if’ analysis, as well as analytical and reporting applications.
Together, SPSS + Planning Analytics can help any industry solve the everyday business problem, enabling line of business employees to make more effective decisions in the forecasting process. SPSS infuses power into the modeling process, and Planning Analytics will build ‘what-if’ analyses to the line of business based on the output from SPSS.
Here is a modeling stream in SPSS using time series models to forecast the demand. From the stream below, the whole data mining process can be covered within SPSS, including data import->data preparation->modeling->evaluation->export.
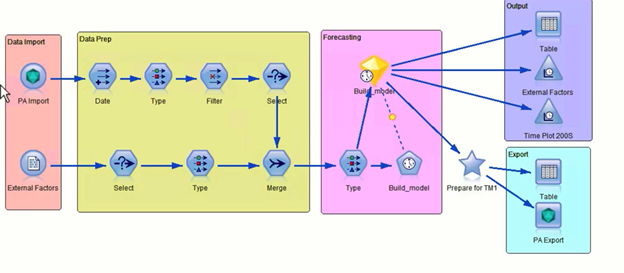
Visualized output from the time series model in SPSS.
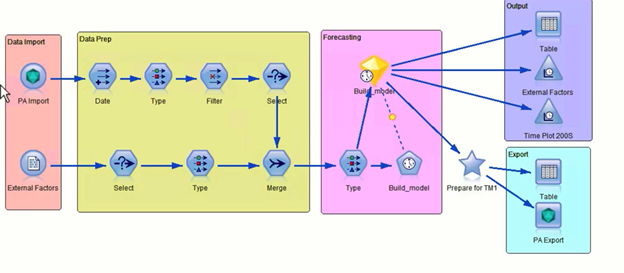
After the model is completed, business users need a visualization layer like a dashboard or report to show values to the stakeholders. In Planning Analytics, users can simply click the button (‘Run Predict Forecast’ in the picture below), to use as a baseline forecast for additional changes in planning analytics, with time series modeling that incorporates external drivers like consumer spending and weather data, it can develop a more reliable forecast with better accuracy.
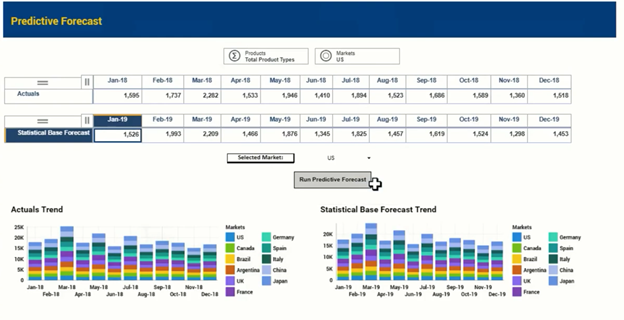
SPSS + Planning Analytics enables users in a range of business functions like marking, product management, and sales to collaborate, and adjust the forecast with their own shared domain knowledge and expertise.