The insurance industry, traditionally seen as a slow and steady sector, is facing new challenges. Climate change, unpredictable customer behavior, and the rise of InsurTech companies are disrupting the status quo.
This blog post explores these challenges and how advanced analytics, specifically Decision Optimization, can help insurance companies navigate this new landscape.
Challenges Faced by the Insurance Industry
The most critical challenges in the insurance industry are:
- Uncertainty in Pricing:Â Unlike most businesses, insurance companies price products (policies) before they know the actual cost (claims). This uncertainty makes it difficult to set the right premium.
- Renewal Price Adjustments:Â Balancing customer retention with increasing revenue is a constant struggle. Offering high renewal prices might push customers away, while low prices can hurt profits.
- Rate Management Complexity:Â Traditional methods of setting rates often lack transparency and consistency, making it difficult to manage changes effectively.
- Asset-Liability Matching:Â Insurance companies need to carefully manage their assets to ensure they can meet future liabilities (claims payouts).
- Life Insurance Product Pricing:Â Complex life insurance products with embedded investment options require careful pricing to ensure profitability and customer satisfaction.
- New Customer Risk Assessment:Â Traditional methods struggle to assess risk for new clients with no claim history.
- Catastrophe Modeling:Â Accurately predicting and managing the financial impact of natural disasters is crucial for insurance companies.
- Optimal Reinsurance Strategies:Â Determining the optimal amount of risk to retain and reinsure is a complex decision that impacts solvency and profitability.
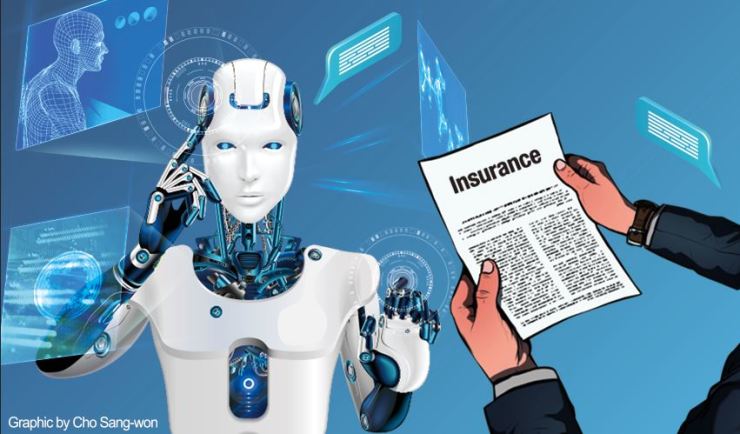
How Decision Optimization Can Help
Decision Optimization is a powerful analytical tool that uses mathematical models and algorithms to find the best course of action under various constraints. Here’s how it can benefit insurance companies:
- Price Optimization:Â By considering factors like customer behavior and competitor pricing, Decision Optimization helps set optimal premiums that maximize profits and customer acquisition.
- Renewal Price Adjustments:Â Decision Optimization models can help find the optimal renewal price for each customer, balancing revenue goals with customer retention.
- Rate Management:Â These models can ensure rate changes are made smoothly and consistently, while adhering to business rules and regulations.
- Asset-Liability Matching:Â Decision Optimization helps find the optimal portfolio of assets that best matches the company’s future liabilities.
- Life Insurance Product Pricing:Â It can determine the optimal minimum guarantee rate, participating rate, and premium for life insurance products with embedded investment options.
- New Customer Risk Assessment:Â Decision Optimization models can leverage various data sources to assess risk for new clients, even without claim history.
- Catastrophe Modeling:Â By integrating catastrophe modeling with Decision Optimization, insurers can make informed decisions about coverage, premiums, reinsurance, and mitigation strategies.
- Optimal Reinsurance Strategies:Â Decision Optimization helps determine the optimal mix of retained risk and reinsurance to maximize profitability and solvency.
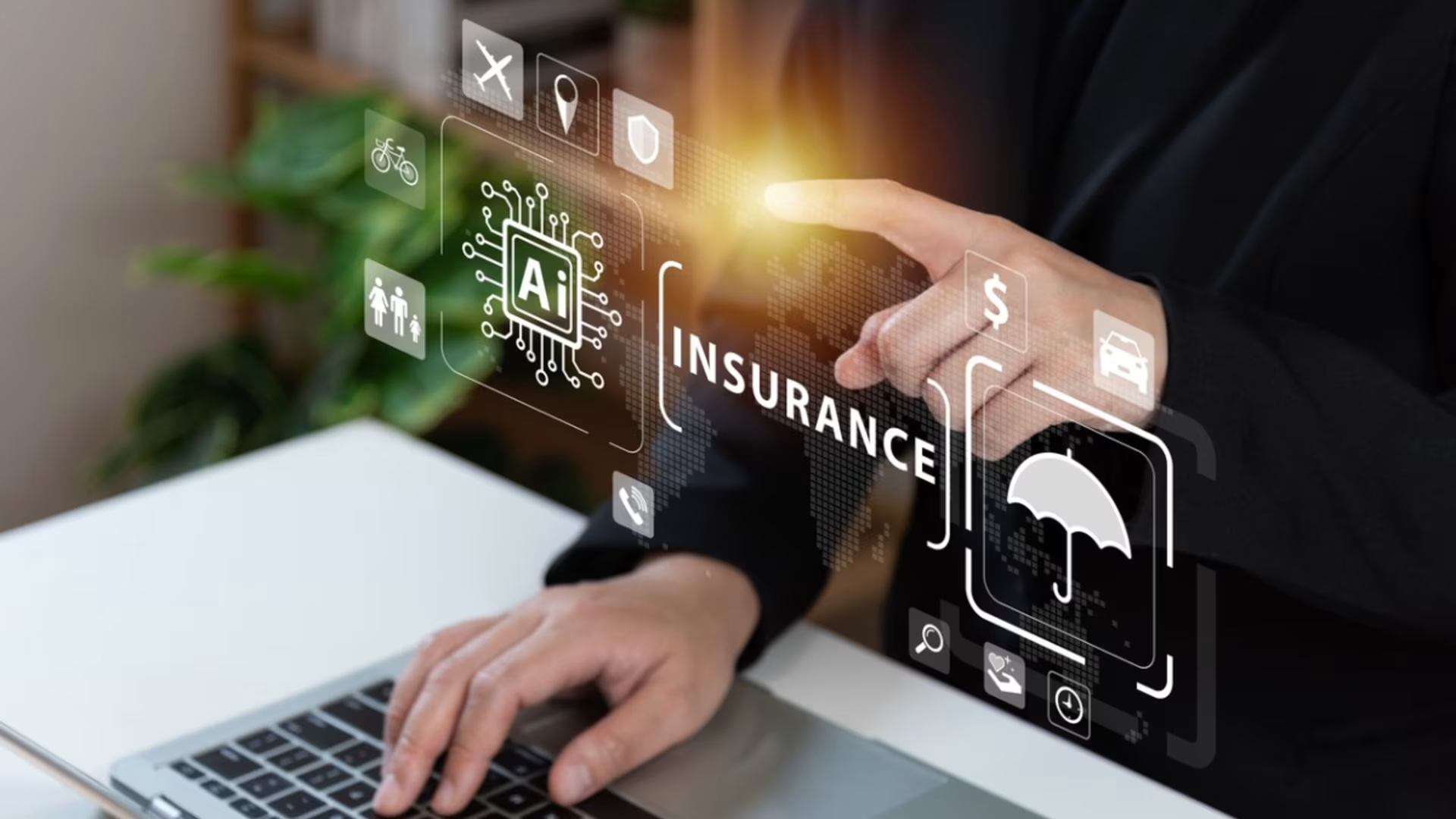
The Benefits of Decision Optimization
The advantages of applying Decision Optimization in the insurance industry are manifold:
Increased profit margins and business volumes.
Higher customer loyalty and retention rates.
Enhanced conversion ratios for desired classes.
Automation of decision-making processes, reducing guesswork and increasing efficiency.
The Road Ahead
The insurance industry is at a crossroads. By embracing new technologies like Decision Optimization, insurers can overcome the challenges of the digital age and position themselves for success in the years to come. Decision Optimization is not a silver bullet, but it is a powerful tool that can help insurance companies make better decisions, improve their bottom line, and ultimately serve their customers more effectively.
Getting Started with Decision Optimization
For insurance companies interested in learning more about Decision Optimization, there are a number of resources available. Many software vendors including IBM, Gurobi, and FICO offer Decision Optimization solutions such as CPLEX, Gurobi Optimizer, FICO Xpress, and FICO Insight specifically designed for Decision Optimization. Additionally, there are a growing number of consultancies that specialize in helping insurance companies implement these solutions. Cresco International is one of the best consulting companies in the area of Decision Optimization that partners with IBM, Gurobi, and FICO.
By taking the time to explore Decision Optimization, insurance companies can unlock a new level of performance and efficiency. In a rapidly changing marketplace, those who embrace innovation will be the ones who thrive.