Ships and ports, planes and airports, trains and railway stations, trucks, and public transportation systems have different cost characteristics, different levels of flexibility, and different planning horizons. Therefore, each mode of transportation has its own set of characteristics and requires Optimization to operate more efficiently and cost-effectively. In this article, we will elaborate on various Optimization use cases for different transportation modes.
1. Optimization for Multimodal Transportation Systems
In intermodal transportation systems, a sufficient number and mix of resources such as trucks, trailers, containers, trains, vessels, etc. are required to meet the freight demands of the customers. Thus, intermodal fleet planning arises as an important issue for logistics companies, which provide multimodal transportation services. Fleet planning consists of various interrelated sub-problems at strategic, tactical, and operational decision levels under the responsibility of multiple decision-makers. We can develop Optimization models to help decision-makers of intermodal transportation systems make optimal decisions in the following aspects.
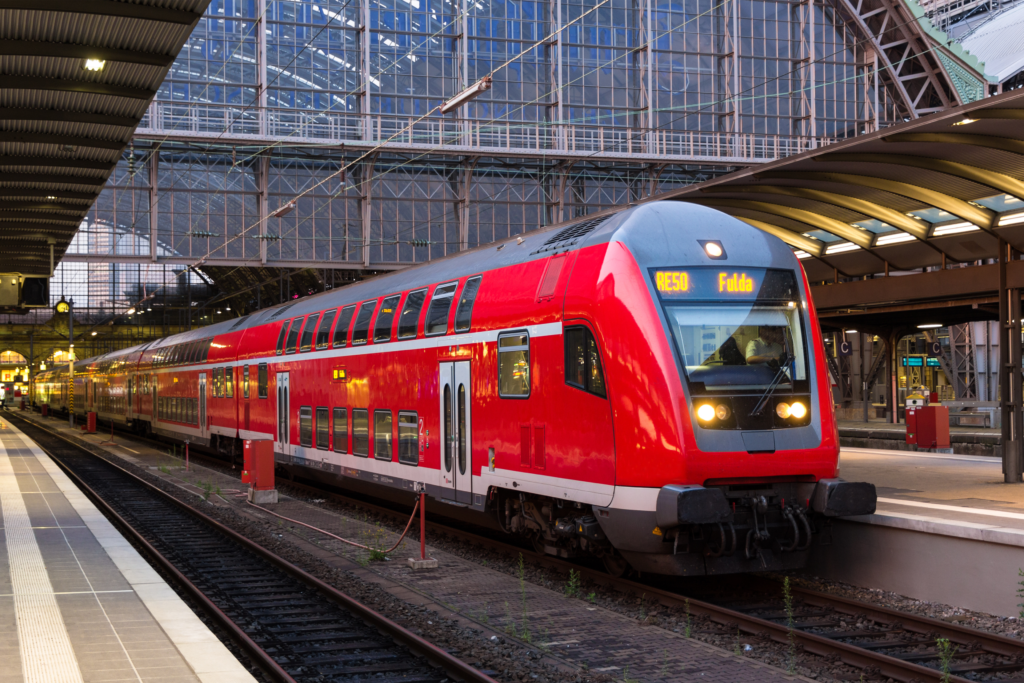
1.1. Long-term fleet planning decisions
- Fleet size optimization: The fleet sizing problem is defined as matching the supply and demand to determine the optimal number of vehicles in the fleet.
- Fleet composition for multiple types of resources
- Fleet expansion, reduction, and replacement decisions: Fleet expansion/reduction deal with the capacity growth and shrinkage of the available fleet size with respect to increase or decrease in transportation service demands, the company’s growth rate, and investment strategies.
1.2. Medium-term fleet planning decisions
- Loaded vehicle movements, vehicle allocations to different transport routes
- Fleet deployment and fleet assignment: Fleet deployment problem is concerned with the optimal allocation of different types of vehicles available in the fleet according to the set of pre-determined routes. The fleet deployment also includes determining the sequence of trips to be performed by each vehicle.
- Planning the number of vessel and train trips
- Service type selection
- Timetable design of trains and Ro-Ro vessels
- Outsourcing or leasing: In intermodal fleet planning, logistics companies may utilize other logistic providers’ resources as an outsourcing option as well as their own fleet of vehicles.
1.3. Short-term fleet planning decisions
- Empty vehicle repositioning: Although empty vehicle repositioning does not contribute to transportation profit directly, it is able to decrease the fleet size and increase the customer service level by decreasing waiting times.
- Load consolidation/exchange at hubs and transshipment terminals: Consolidation of loads according to their spatial attributes and time windows to the appropriate vehicles and routes is one of the most promising operational decisions in intermodal transportation. By combining the different loads according to their spatial attributes and time windows to the appropriate vehicles and routes at the hubs and terminals, transportation costs can be decreased significantly.
- Itinerary re-planning and rescheduling transport capacity based on real-time data
- Vehicle inventory management: We should consider initial vehicle inventory levels, outgoing, and incoming loaded vehicle movements, empty vehicle repositions, and vehicle purchases/sales to compute the periodic vehicle inventories.
- Vehicle routing, train, and vessel scheduling.
2. Optimization for Public Transportation
2.1. The five stages for designing a public transportation system are route network design, frequency setting, timetable design, fleet assignment, and crew assignment. In real systems, usually, these stages are performed sequentially, where decisions taken at a given stage influence decisions taken at subsequent stages. The frequency setting problem determines the time interval between subsequent buses on the lines, based on their itinerary and the demand given by an origin-destination matrix. When designing the itinerary of the lines, i.e., the route network, a preliminary setting of frequencies is needed. Also, during the tactical planning it is necessary to adjust the frequencies to demand variations along different seasons of the year or time of day, or as response to changes in the route network design. The frequencies impact on both the users (waiting time, the capacity of the lines) and also the operators (operational cost determined strongly by the size of the required fleet).
We can develop transit frequency optimization models to determine the time interval between subsequent buses for a set of public transportation lines given by their itineraries, i.e., sequences of stops and street sections. We should include origin-destination demand constraints, constraints on the available fleet of buses, and other infrastructure and policy constraints in these optimization models. We can define the objective of these Optimization models as the minimization of the overall travel time of the users (walking, onboard, and waiting).
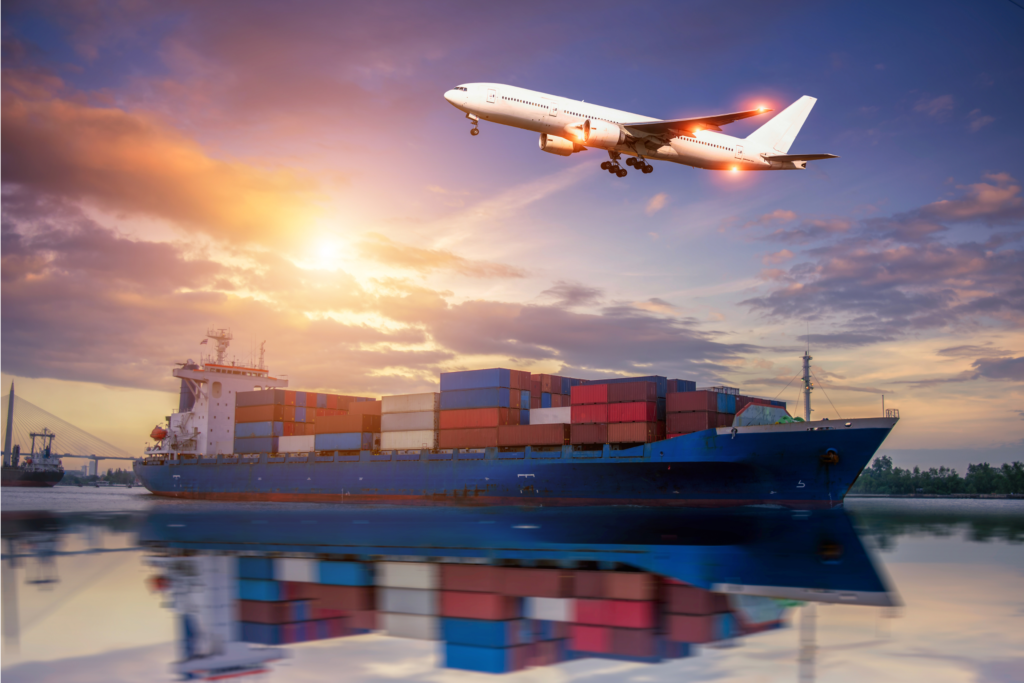
2.2. An intermodal public transportation network is defined as a set of train, metro, tramway, or bus lines, i.e., the vehicles moving between different stations. Passengers traveling in public transportation networks often have to use different lines to cover the trip from their origin to the desired destination. Thus, the reliability of connections between vehicles is a key issue for the attractiveness of the intermodal transportation network and it is strongly affected by some unpredictable events like breakdowns or vehicle delays. For example, if a vehicle is delayed, the users of this vehicle who want to transfer to another vehicle at a station could miss their connection. Â To avoid passengers missing their transfers, one could force all departing vehicles to wait until the delayed one has arrived. But, in this case, the delay spreads out through the network, thus affecting many customers. On the other hand, if all vehicles depart on time, no other passengers but the ones on the delayed vehicle will be concerned: the number of affected passengers is thus minimized but they will suffer greater delays as they miss their connections. As a consequence, the best decision is generally to force only a subset of the vehicles to wait for the delayed ones.
We can apply delay optimization models to the public transportation systems to determine the optimal wait/depart policy that minimizes the total delay on the network. The delay optimization models determine if the connected vehicles should wait for the delayed ones or keep their schedule. In other words, these Optimization models determine how other vehicles of the network should react (wait or no wait) in order to minimize the sum of delays of all the passengers at their destinations.
3. Optimization for Railway Transportation Systems
3.1. In a railroad system, train pathing is concerned with the assignment of trains to links and tracks, and train timetabling allocates time slots to trains. We can develop train timetabling optimization models to determine the optimal times of arrival and departure of a train at all stations.
3.2. We can develop railway crew scheduling optimization models to find the most efficient duty combination for railway crews to cover all trains and related activities for a defined period of time. Decisions on railway crew members can be classified into different planning levels. At a strategic and tactical level with a time horizon longer than one year, crew planning deals with the long-term availability and capabilities of crew members as well as the location and the capacities of crew depots (crew management). For reliable long-term operations, it is crucial to anticipate the future need of different crew types and to realize a balanced crew composition regarding age and skills. This includes decisions on hiring, training, or moving crews from one depot to another. Besides, the choice of crew depot locations including opening or closing decisions play an important role at this stage. At the operational level, crew planning is the task of building work schedules for crew members to operate a planned timetable.
4. Optimization for Municipal Solid Waste Collection
4.1. Optimization of municipal solid waste collection and transportation through source separation becomes one of the major concerns in the municipal solid waste management system design, due to the fact that the existing municipal solid waste management systems suffer from high collection and transportation costs. Generally, in a city, different waste sources are scattered throughout the city in a heterogeneous way which increases waste collection and transportation costs in the waste management system. Therefore, the shortest waste collection and transportation strategy can effectively reduce waste collection and transportation costs.
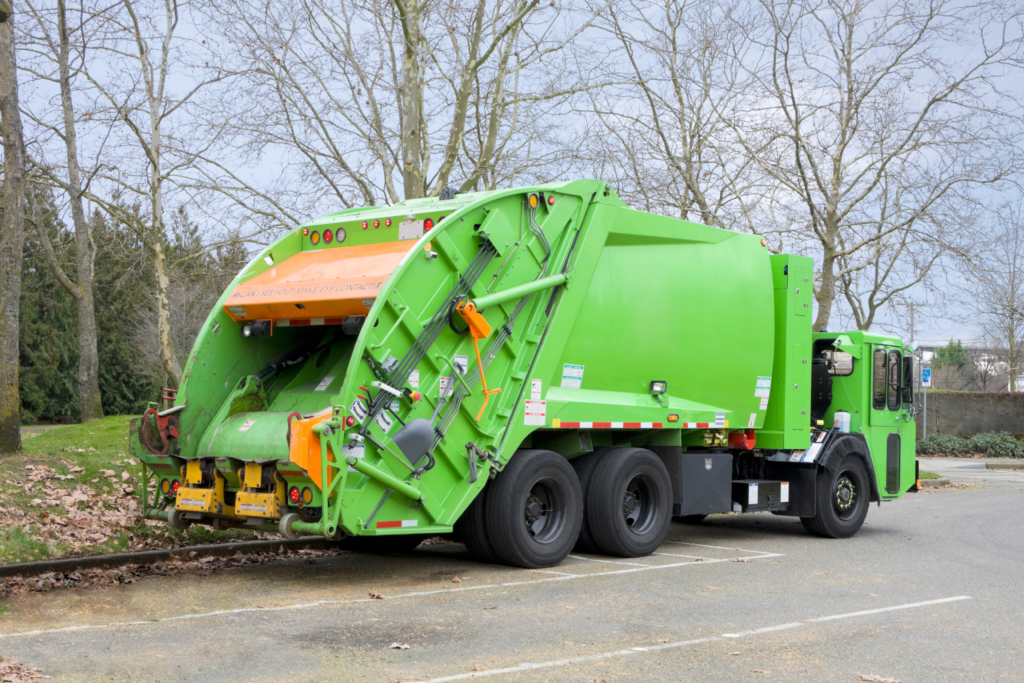
Municipal solid waste management is a multidisciplinary activity that includes generation, source separation, storage, collection, transfer and transportation, processing and recovery, and disposal. From an Optimization perspective, we can focus on the collection, transfer, and transportation of solid waste from any waste generation sources, such as households, markets, institutions, and offices, to the processing plant or landfill site. We can propose an optimal municipal solid waste collection and transportation scheme that focus on the problem of minimizing the length of each waste collection and transportation route. In our optimal scheme, we can divide the whole integrated waste management system into the four following parts: (i) Collection of segregated solid waste from various sources) and conveyed waste to the nearest collection center; (ii) transfer accumulated solid waste from the collection center to the adjoining transfer station; (iii) transport the segregated solid waste from the transfer station to a nearby categorized waste processing plant; (iv) transfer the produced waste from the processing plant to the nearest landfill site. As each part contains a huge amount of cost for waste collection or waste material transportation, our proposed scheme can optimize the transportation cost of each part of the system. Therefore, the total waste management cost for the whole system is optimized. For example, we can develop Travelling Salesman Problem (TSP) Optimization models to optimize municipal solid waste collection and transportation routes.
5. Optimization for Shipping Companies
A ship involves a major capital investment (usually millions of U.S. dollars), and the daily operating costs of a ship can be tens of thousands of dollars. This means that improving fleet utilization can be translated into significant improvements in financial results. Another positive result of increasing fleet utilization can be reduced damage to the environment because of reductions in transport operations. It is clear from the above that there is a considerable need for, and potential benefits from, Optimization decision support systems in ship scheduling.
We can develop different types of Optimization models for making optimal strategic, tactical, and operational decisions. For example, we can develop Optimization models to determine the optimal size and mix of the fleet, routing and scheduling for each ship in the fleet, and selecting the best course for a ship between two ports.
Ship routing and scheduling problems are different from those of other transportation modes because ships operate under different conditions. For example: (1) ships pay port fees, (2) the draft of a ship is a function of the weight of the load (affects ship-port compatibility), (3) ships operate mostly in international trade (crossing multiple jurisdictions), (4) ships can be diverted at sea, and (5) ship voyages span days or weeks and their time in port may cover several port operating time windows.
The following list reports some of the most important use cases of Optimization for shipping companies that we can offer to our clients:
- Optimization models for minimizing the number of tankers needed to perform a given set of schedules. This can be considered a fleet sizing problem in which there is only one type of vessel.
- Optimization models for determining optimal liner routes for a container shipping company.
- Optimization models for determining the optimal number of ships and fleet deployment plan in a containership hub and spoke application
- Optimization models for berth allocation and planning
- Optimization models for container stowage planning
- Optimization models for crane scheduling
- Optimization models for fleet sizing and routing for straddle carriers
- Optimization models for scheduling of ships’ arrivals at ports to minimize demurrage
- Optimization models for a decision-support system for allocating crude oil loads of tanker ships to port and refinery tanks
- Optimization models for scheduling of harbor pilots
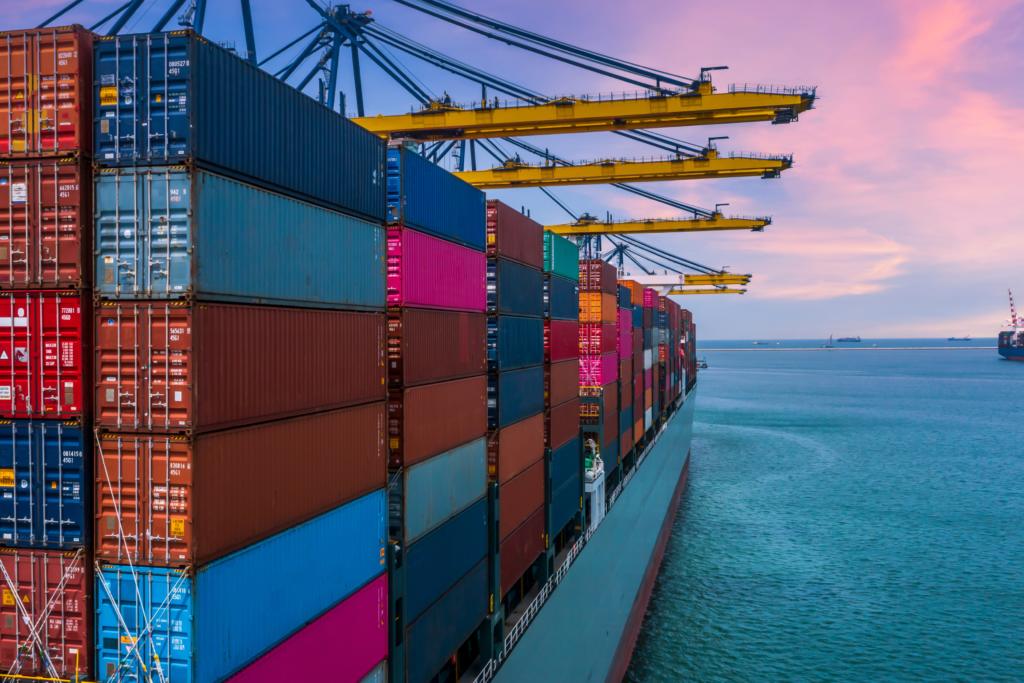
Let’s consider Optimization models for vessel scheduling in liner shipping as an example. Liner shipping plays a major role in freight transportation and international seaborne trade. One of the most challenging decision problems, tackled by liner shipping companies, is the design of vessel schedules. At the vessel scheduling stage, the liner shipping company aims to determine vessel sailing speeds at voyage legs of a given liner shipping route, port times, vessel handling rates at ports, the minimum number of vessels required in order to provide the agreed service frequency at ports, and other factors. Liner shipping companies deal with three levels of decision problems and we can develop Optimization models for each level of decision making. The strategic-level decisions consist of long-term decisions, such as vessel fleet size and mix, alliance strategies, and network design. Tactical-level decisions include determination of the frequency of liner shipping services, deployment of vessels on the respective port rotations, determination of vessel sailing speeds, and design of vessel schedules. The operational-level decision problems include cargo booking, cargo routing, and vessel rescheduling due to disruptions, such as congestion at ports, adverse weather, malfunctioning of vessel engines, and others.
6. Cross-Dock Scheduling
Cross-docking is typically described as a logistic procedure, where the products are transferred by the inbound trucks from a supplier or a manufacturing plant to a cross-docking terminal. Then, the products are deconsolidated, sorted based on the customer’s preferences, consolidated, and shipped out to the end customers using the outbound trucks. Accordingly, a cross-dock is a consolidation point in a distribution network.
We can develop Optimization models for the following challenges of a cross-docking terminal:
- Location of the cross-docking terminals
- The layout of the terminal
- Assignment of destinations to dock doors
- Vehicle routing
- Truck Scheduling
- Resource scheduling inside the terminal
- Packing loads into trucks
- Unpacking loads from trucks
As an example, we can develop Optimization models for truck scheduling, which decides the succession of truck processing at the dock doors. These Optimization models are necessary to ensure a rapid turnover and on-time deliveries.
7. Charge Scheduling of Electric Vehicles in Smart Grid
The number of electric vehicles is expected to grow rapidly in the coming years. However, the uncoordinated charging of these vehicles can put severe stress on the power grid. The problem of charge scheduling of electric vehicles is an important and challenging problem. The electric vehicle charge scheduling problem takes as input a set of electric vehicles and different grid, user, and aggregator-side parameters and outputs a charging schedule (starting and ending times of charging of each electric vehicle in the set). It is an optimization problem that optimizes some grid, user, or aggregator-side parameters (or a mix of them) subject to the different grid, user, and aggregator-side constraints. The parameters, constraints, and objective functions considered span a very wide range, giving rise to different forms of optimization problems.
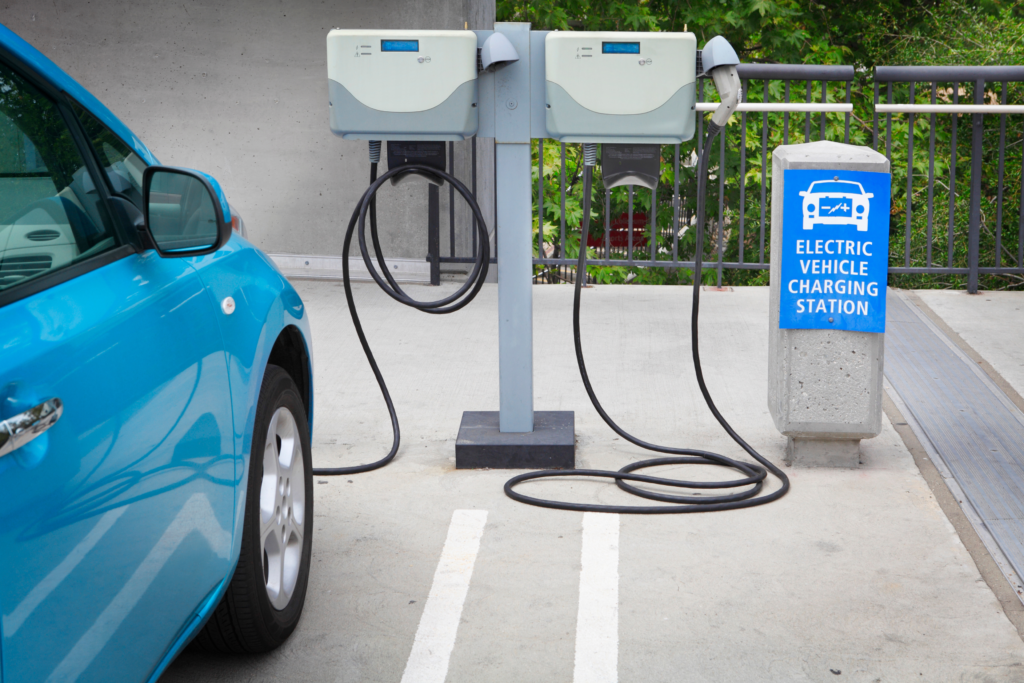
We can develop Optimization models with different objective functions depending on the goals of scheduling. For example, we can optimize grid-side benefits such as minimizing the financial cost of power supply, minimizing grid operation cost, including the cost of wind and hydropower and availability cost for providing spinning reserves, minimizing distribution system load variance, minimizing loss in the distribution system, and maximizing the profits of thermal and wind plants while minimizing energy trading risks. In another example, we can optimize electric vehicle-side benefits such as minimizing charging cost, minimizing both CO2 emission and charging cost, and maximizing user convenience. Moreover, we can focus on optimizing aggregator-side benefits, such as maximizing aggregator profits and reducing the imbalances arising out of the energy purchased by the aggregator from the day ahead.
8. Optimization for Forest Industry
Transportation of forest products accounts for a major contributor to the total operational costs. For example, the transportation cost of logs from forest sites to mills represents a major expense and accounts for more than 45% of the total operational cost in some countries Additionally, transportation costs could account for 50% of the total cost of delivered biomass to bioconversion plants in some cases, which makes transportation a key factor affecting the viability of the bio-industry. Therefore, transportation is an important aspect of supply chain planning, and even a small reduction in the transportation cost could lead to large savings.
We can develop Optimization models for transportation decision-making at the operational level including decisions related to product flow, storage, pre-processing, and routing and scheduling of vehicles. In our Optimization models, we can focus on the transportation of different products such as logs, biomass, pulp, and furniture. We can focus on optimizing the total cost of transportation, the environmental aspects of truck routing and scheduling in forestry, and pre-processing (e.g., sorting, grinding, blending, bucking) decisions at forest sites, satellite yards, and mills.
The three main types of transportation problems in forestry are: (1) destination problems; (2) backhauling; and (3) daily/weekly truck scheduling and dispatching problems. We can develop Optimization models for each of these problems.
9. Optimization for Truck Transportation of Hazardous Materials
The transport of hazmat on public roads poses a direct threat to the resident population and environment. Therefore, the route optimization of hazardous material transportation is not only a sensitive public safety problem, but also an important strategic decision-making problem. Hazardous materials are referred to those substances and articles with explosive, inflammable, toxic, infectious, corrosive, and other dangerous characteristics that are liable to cause human casualties, property damage, or environmental pollution in the course of production, management, transportation, storage, use, and disposal, which is required special protection. Â Truck routing offers a lot of optimization possibilities. For example, we can develop different types of Optimization models for the following problems:
- Shortest path problems without scheduling
- Shortest path problems with a priori scheduling
- Shortest path problems with adaptive route selection
- Dissimilar path problems
- Vehicle routing problems
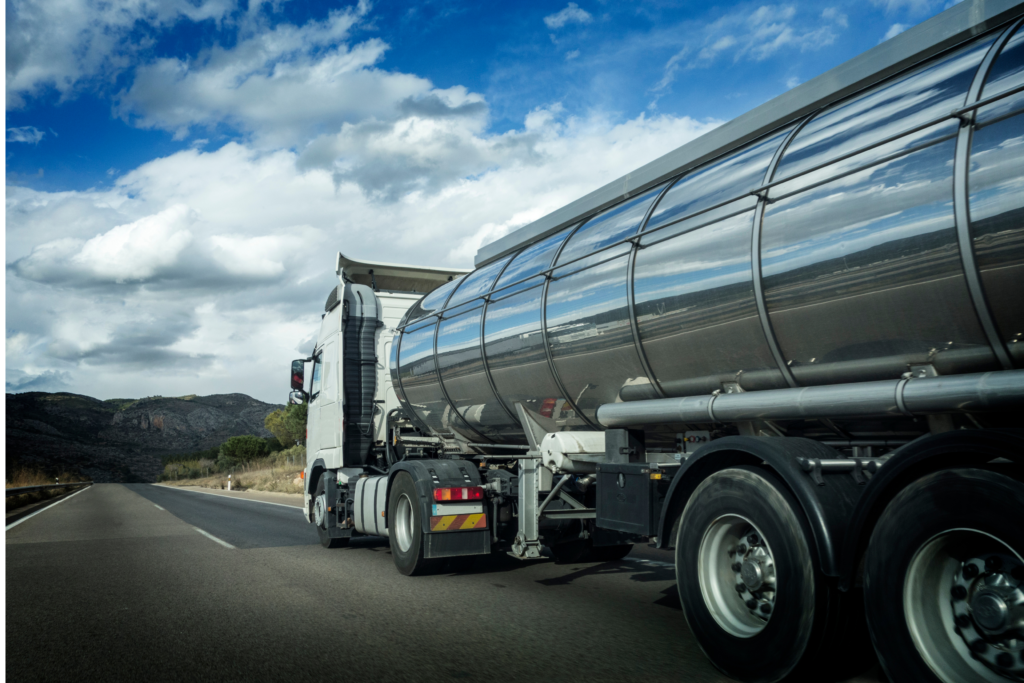
ÂWe can consider different types of objectives for these Optimization models. Also, we can consider multiple objectives at the same time. For example, we can consider objectives like minimizing risk, minimizing accident rate, and minimizing operating cost at the same time in one Optimization model.
10. Optimization for Airlines
10.2. Airport runway optimization is an ongoing challenge for air traffic controllers. Since demand for air transportation is predicted to increase, there is a need to realize additional take-off and landing slots through better runway scheduling.
One of the main factors that determine the runway throughput at an airport is the required minimum separation between aircraft during landing and take-off. The dependency of separation on the leading and trailing aircraft type makes the sequencing and scheduling of landings and take-offs an important and non-trivial problem. Careful sequencing and scheduling can reduce the number of long separation times thereby opening up opportunities for new landing or takeoff slots.
The Aircraft Landing Problem and the Aircraft Take-off Problem seek to determine the sequence of aircraft landing on or taking off from, the available runways at airports in order to optimize given objectives, subject to a variety of operational constraints. Scheduling of the landing and take-off of aircraft can be divided into three stages: creating an initial schedule, modifying the schedule, and freezing the schedule.
10.1. A significant problem faced by every airline is constructing a daily schedule for a heterogeneous aircraft fleet. A plane schedule consists of a sequence of flight legs that have to be flown by plane with the exact times at which the legs must start and finish at the respective airports. The first part of the problem (determining the sequence of flight legs) is basically a routing problem. In contrast, the second part of the problem determining the exact times) is a scheduling problem. The fleet schedule is important since the airline’s total revenue can be estimated if the demand function of each leg is known. Moreover, the fleet schedule also determines the total cost incurred by the airline, including the fuel cost and the crews’ salaries.
Given a heterogeneous aircraft fleet, a collection of flight legs that have to be flown in one day with departure time windows, durations, and cost/revenues corresponding to the aircraft type for each leg, a fleet schedule has to be generated that maximizes the airline’s profits (possibly subject to certain additional constraints).
In conclusion, optimizing transportation systems can lead to more efficient and cost-effective operations, which ultimately benefit both businesses and consumers. Each mode of transportation has its own unique set of characteristics, including cost, flexibility, and planning horizons. As a result, transportation decision-makers must utilize Optimization models to make optimal decisions in various aspects of fleet planning, including long-term fleet planning decisions, medium-term fleet planning decisions, and short-term fleet planning decisions. For intermodal transportation systems, fleet planning is especially important, as it involves a sufficient mix of resources, such as trucks, trailers, containers, trains, and vessels, to meet customer demands. In addition, public transportation systems require a complex set of optimization strategies, including transit frequency optimization and delay optimization, to improve the overall travel time and reliability of the system. By leveraging optimization models, transportation decision-makers can make informed decisions that will ultimately lead to better transportation systems for all.