Competition within the healthcare industry is on the rise. To stay in the market, healthcare organizations face the challenge of reducing operational costs while maintaining, if not improving, the quality of patient care and services provided. One of the tools that can help healthcare managers reach their goals is optimization. Indeed, they can use optimization to identify their issues and explore the right solutions to fit their goals. For example, implementing optimization techniques in hospitals can result in financial returns on investment that range between $17,000 and $300,000/bed/year coupled with improved quality of care. In what follows, first, we will talk about the role of optimization in improving patient flow in medical centers. Then, we will elaborate on the most critical use cases of optimization in healthcare scheduling. Next, we will discuss the prominent use cases of optimization in the supply chains of healthcare providers. Finally, we will introduce a couple of optimization use cases in medicine.
1. Patient Flow Optimization
Healthcare providers such as hospitals and clinics might experience any of the following issues due to improper allocation of resources (e.g., exam rooms, nurse practitioner staffing, beds) across units in response to clinically-driven random arrivals of patients:
- Extensive emergency department boarding, including overnight holds
- Crowded surgical units, especially on weekends
- High or low occupancy units
- Hallway placement of inpatients
- Bottleneck units
- High readmission rates to particular units
- The excessively long length of stay, particularly in higher levels of care
We can use optimization techniques to improve hospital-wide patient flow by uncovering bottlenecks and identifying their sources and impact, leading to impressive results in quality improvement and cost reduction. Indeed, these techniques allow healthcare managers to make informed judgments on the resource capacity needed to serve variable demand flows in different medical services. Â For example, the queuing models make it possible for hospitals to predict and manage the variability of random patient demand. Our optimization models can help hospitals improve in the following aspects by adequately allocating the resources like beds across units and optimizing staffing to achieve the desired service:
- Eliminating unnecessary peaks and valleys in their patient flow
- Increasing the number of patients that can be treated
- Reducing nursing overload, overtime, and turnover
- Achieving maximum compliance with patient-per-nurse ratios without hiring new staff
- Assuring patients are placed in the correct beds
- Improving patient, doctor, and nurse satisfaction
- Improving the quality and safety of patient care
- Reducing patient wait times for services
- Reducing hospital overcrowding
- Lower overall hospital length of stay
The following statistics show the success of applying patient flow optimization to some hospitals in the U.S. by other consulting companies:
- Annual staff costs reduced by: $1.07 million in Mayo Clinic, $ 1 million in Greater Baltimore Medical Center, $130,000 in Boston Medical Center
- Waiting time decreased by: 2 hours in Boston Medical Center (emergency department), 34% and 84% in Overlook Medical Center (discharge and transfer in the ICU, respectively)
- The average length of stay decreased by: 14% in Overlook Medical Center
- The number of treated patients increased by: 25% at St. Thomas Community Health Center
- Capital costs decreased by: $ 2 million in Greater Baltimore Medical Center (by opening needed medical beds rather than building telemetry beds), $10 million per year in Newark Beth Israel Medical Center (by eliminating 26 telemetry beds)
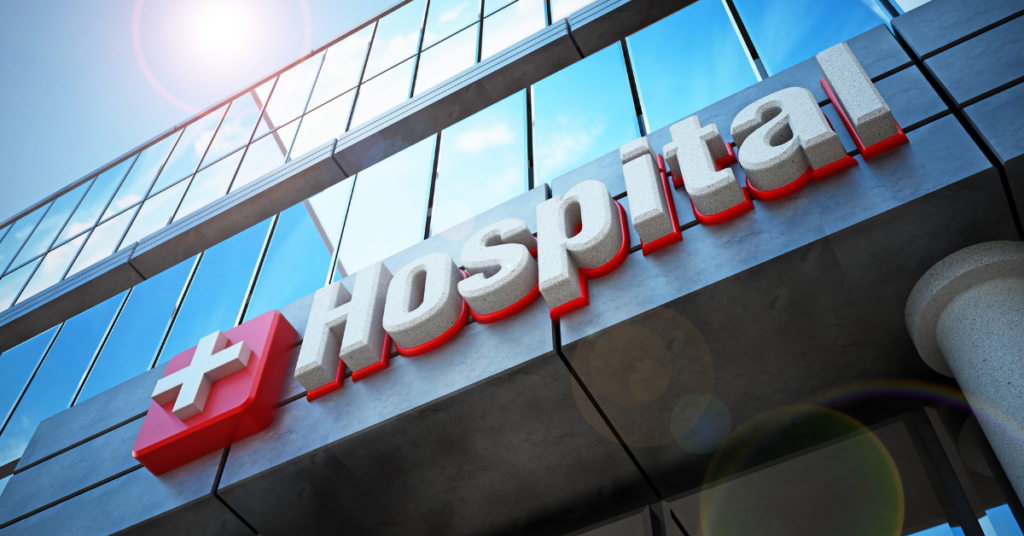
2. Optimization in Healthcare Scheduling
The inability to align hospital capacity with patient demand for services results in system stress, widespread waste, and inefficiency. Suboptimal scheduling practices frequently cause hospital overcrowding, readmissions, medical errors, hospital-acquired infections, delays, lack of preferred beds, cancellations, underutilization of existing resources, inflated cost, and nurse and physician burnout. The traditional solutions of adding more physical capacity or increasing staffing are no longer feasible (or advisable) in today’s healthcare environment. Optimization can assist healthcare providers in optimizing their efficiency, rapidly generating revenue, and improving quality without trade-offs.Â
2.1. Operating Room Scheduling Optimization
More than 60% of hospital admissions are surgical operations and operating rooms compete for resources with many other departments. Accordingly, hospitals may experience any of the following issues caused by inefficient scheduling of their operating rooms:
- Difficulties in accessing the operating rooms for urgent and emergent surgeries
- A significant number of urgent and emergent surgeries done after prime-time hours (facility’s business hours)
- Significant moving and delaying of elective surgeries to accommodate urgent and emergent surgeries
- High operating room staff turnover that results in operating room chaos and staff dissatisfaction
- The increased waiting time for the operating room for urgent and emergent surgeries
- Inability to grow surgical volume because of perceived operating room capacity limitation
We can use optimization techniques to address these issues by dividing three levels of operating room planning and scheduling decisions. At the strategic level, the optimization problems include capacity planning, allocation, and case-mix problems. Capacity planning is the process of determining the number of resources necessary to meet demands cost-effectively. Capacity allocation refers to the allocation of specialties to operating-room days in the operating room management. Case-mix planning assigns the operating room time blocks among the surgical specialties in a long-term time horizon. We can consider common objectives in our operating room scheduling optimization problems, such as minimizing the idle times of the operating rooms and minimizing the times surgeons have to wait for an operating room to become available. At the tactical level, we can consider a cyclic operating room scheduling optimization problem called the master surgical scheduling problem. At the operational level, our optimization problem is a surgery scheduling optimization problem, which determines the scheduled date, time, and specific required resources allocated to certain surgical cases, including advance scheduling, allocation scheduling, and integration of these two steps.
Our operating room scheduling optimization techniques can bring the following benefits to hospitals:
- Shorter waiting times for surgery for emergent and urgent patients
- Reducing or eliminating cancellations and postponement of elective surgeries
- Reducing operating room overtime
- Higher operating room prime-time utilization
- Increasing surgical volume
- Reducing cost per surgical case
- Reducing inpatient length of stay
- Improving patient safety by reducing hospital-acquired infections and mortality
- Improving patient and healthcare provider satisfaction
The following statistics showcase the success of applying operating room scheduling optimization techniques to some hospitals in the U.S. and Canada by other consulting companies:
- Surgical volume increased by: 3,000 cases in University Health Network, 4% in Mayo Clinic, 5% in Cincinnati Children’s Hospital Medical Center, 10% in Boston Medical Center, 3% in Palmetto Richland Memorial Hospital
- Operating room overtime decreased by: 27% in Mayo Clinic, 57% in Cincinnati Children’s Hospital Medical Center, 6.6% in The Johns Hopkins Hospital
- Operating room utilization increased by: 5% in Mayo Clinic, 20% in Great Ormond Street Hospital for Children
- The waiting time for surgeries was reduced by: 20% in University Health Network, 28% in Cincinnati Children’s Hospital Medical Center, 39% in the Johns Hopkins Hospital, 38% in Palmetto Richland Memorial Hospital
- Annual revenue/cost savings increased by: $5 million in Mayo Clinic, $115 million in Cincinnati Children’s Hospital Medical Center, $4 million in the Johns Hopkins Hospital, $8 million in Palmetto Richland Memorial Hospital, $3 million in Greater Baltimore Medical Center
- The canceled surgeries were reduced by: 600 per year in the Ottawa Hospital, 5% in Boston Medical Center, 2.3% in Great Ormond Street Hospital for Children
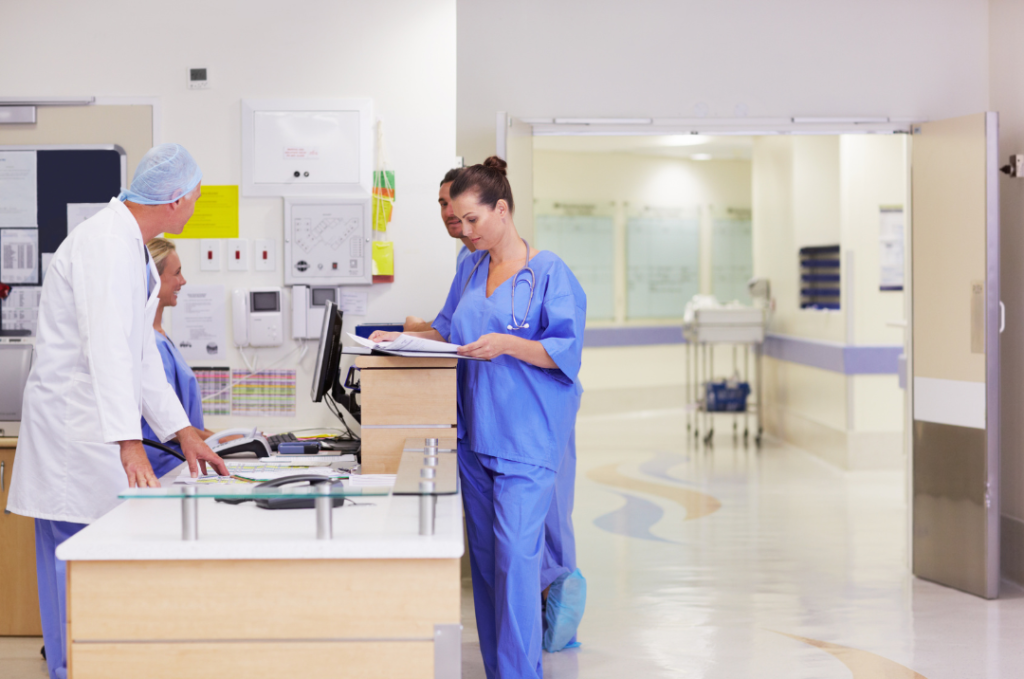
2.2. Physician Scheduling Optimization
Physicians are one of the most expensive resources in hospitals and are considered the bottleneck of the care-providing process. Physicians typically have individually negotiated agreements with their employers, which leads to a partial transfer of power from management to physicians and the importance of adhering to the physicians’ schedule preferences and contractual agreements for managers.
We can use optimization techniques to optimize physicians’ schedules based on their preferences and fairness issues. The main objectives that we can consider in our physician scheduling optimization problems can either be financial or non-financial. For example, we can express financial goals in monetary units derived from the hospital’s economic incentives. For non-financial objectives, we can focus on staff-related measures that include working conditions such as the reduction of overtime hours and different fairness aspects such as equal distribution of workload, unpopular shift types, or weekends off.
2.3. Catheterization Laboratory Scheduling Optimization
A catheterization laboratory is an examination room in a hospital or clinic with diagnostic imaging equipment. Different patient streams compete both for catheterization laboratory access and downstream resources, such as telemetry beds. Therefore, catheterization laboratories may experience several problems, such as long waiting times, cancellation of procedures, high overtime, poor utilization, low throughput, high cost per procedure, and poor access to telemetry beds, that affect other services with whom they directly interact (e.g., telemetry units) or with whom they compete for hospital resources (e.g., the emergency department).
We can obtain optimal schedules for catheterization laboratories using optimization models that satisfy unique requirements of their procedures, such as urgency, duration of the procedure, and need for downstream resources. With our optimization models, we can alleviate the mentioned issues by bringing the following benefits to catheterization laboratories: reducing patients’ waiting times for placement in an inpatient bed, improving access to telemetry beds, increasing throughput, decreasing overtime, and reducing refusals of transfers requiring catheterization laboratory access.
2.4. Nurse Scheduling Optimization
Nurses are one of the essential resources in the U.S. healthcare system. They account for approximately 25% of the total hospital operating budget and 44 % of direct care costs. However, healthcare managers face several challenges in managing this vital resource efficiently. For example, unsatisfactory nurse scheduling is a significant reason for nurse resignation, cited by 30.4% as the main reason behind their resignation. More than 50% of full-time nurses work an average of seven hours of overtime each week, which has caused only 25% nurse satisfaction in this country. We can use nurse scheduling optimization to help healthcare managers fix or alleviate such problems.
Nurse scheduling optimization assigns an optimal number of skilled nurses to each shift to satisfy the demand of a hospital. We can use optimization to minimize the overall hospital cost and maximize nurses’ preferences while considering the governmental regulations, labor laws, hospital policy, and the status of nurses. The following statistics indicate the success of applying nurse scheduling optimization techniques to some hospitals around the world:
- Improving nurses’ satisfaction up to 34% by creating a fair schedule system
- Decreasing the overall cost up to 11% and overtime cost by 36%.
- Up to a 30% reduction in overtime schedule
- Elimination of an average of 4 hours of manual scheduling
- Reducing the risk of healthcare-associated infections in hospitals up to 27%.
2.5. Home Healthcare Routing and Scheduling Optimization
In home healthcare operations, nurses are scheduled and routed to perform various services at clients’ homes. Home healthcare planners assign nurses to clients and schedule working times. They do routing and scheduling tasks manually, resulting in high efforts and inefficient plans and schedules. For instance, driving time accounts for between 18% and 26% of working time, which indicates the high potential for optimization in routing and scheduling.
We can use a combination of vehicle routing and scheduling optimization approaches to improve operations and lower expenses. Our optimization models can include different decision levels, such as shift scheduling, staff assignment, and staff routing decisions. We can also consider different objectives in our optimization models, such as minimizing the patients’ waiting time, minimizing the idle time of resources, maximizing satisfaction, minimizing the time to complete all tasks, and maximizing the number of visited patients. Besides, We have to consider various requirements, including but not limited to matching nurses’ skills and clients’ requirements, respecting preferences, various regulations, continuity of care, and workload balance measurements. Moreover, we should pay attention to the fact that some home health care services are time-sensitive, e.g., insulin injections have to be administered within certain time frames.
2.6. Radiotherapy Treatment Scheduling Optimization
In oncology departments, the scheduling of radiotherapy for patients plays a crucial role in ensuring the delivery of the proper treatment at the right time. It is well known that a large amount of radiation can be delivered safely to a tumor if it is spread out over several weeks. This procedure saves healthy tissue from unnecessary damage and gives it time to recover. A patient has to visit the treatment center several times a week for a given number of weeks, dependent upon the treatment plan.
We can apply optimization techniques to the scheduling of the treatment plans to improve the radiotherapy treatment efficacy. Our optimization models will deal with the allocation of the patients to treatments over time, subject to all the possible constraints. As an example, we can develop an optimization model that maximizes the number of patients to be scheduled, taking into account the following constraints: (1) the priority level assigned to the patient (which may depend on the “severity” of the patient’s illness); (2) the number of treatment sessions for each patient in a week; (3) the treatment sessions for each patient have to be done on consecutive; (4) each patient has to undergo the treatment for a given number of consecutive weeks.
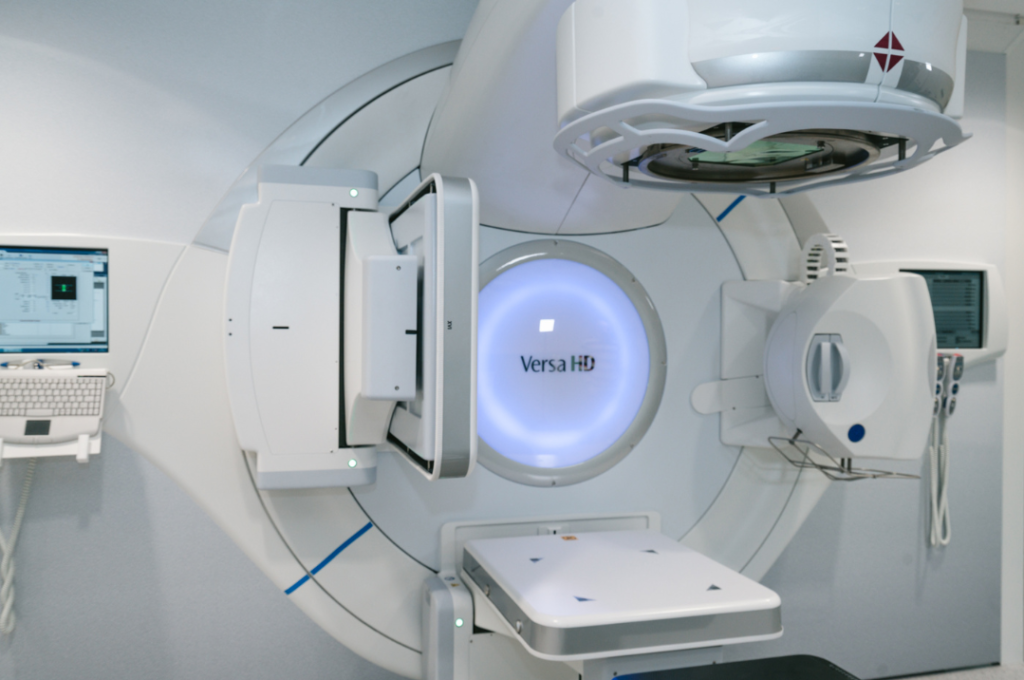
2.7. Patient Multi-appointment Scheduling Optimization
In hospitals, patients need to sequentially visit multiple resource types such as physicians and nurses to receive treatment or be diagnosed. We can consider this process as a multi-appointment scheduling optimization problem. In this optimization problem, we should assign each patient to a specific path over a subset of the considered resources, and each step needs to be scheduled. This optimization problem lets each patient visit the resources in his or her subset of resources within the allotted time to receive timely care. Using this optimization approach, we can lower the risk of delayed diagnosis or treatment, which results in adverse health effects. Besides, our multi-appointment scheduling optimization models can increase patients’ satisfaction, allowing them to visit the hospital less frequently.
It is worth mentioning that we can apply our multi-appointment scheduling optimization techniques to different hospital departments. The scheduling problems that arise in each of these departments are significantly different. For example, in rehabilitation departments, patients recover from physical injuries or drug addictions. Treating patients usually require multiple specialists and devices from several departments. A visit to these resources must be carefully planned, which is often a complicated task involving many human actors. The second application occurs when patients need to be scheduled for diagnostic tests. These tests usually do not take a long time so that patients can undergo multiple tests in a single day. Doing so allows doctors to diagnose patients faster. Most of this subset of manuscripts aims to minimize the completion time of all steps in the care chain. We can apply our optimization models to the oncology department as well. In these departments, we can mainly focus on scheduling patients on the chemotherapy chairs or linear accelerators (used for radiotherapy) and the scheduling process for the entire care pathway, including consultations with oncologists and pre-treatment stages.
3. Optimization in Healthcare Supply Chain
The healthcare supply chain consists of several product types and the contribution of different stakeholders, including producers (e.g., medical and surgical devices, pharmaceuticals), purchasers (e.g., wholesalers, distributors), and providers (e.g., hospitals, physicians, clinics, pharmacies, nursing homes). The supply process begins with the manufacturer and finishes with the healthcare provider’s patients. The main goal of the healthcare supply chain is to provide products at the right time and fulfill the requirements of healthcare service providers. In what follows, we will elaborate on three optimization use cases in healthcare supply chains.
3.1. Pharmaceutical Inventory Optimization
The inventory of pharmaceuticals within the hospital pharmacy is complex. For example, there may be as many as 2,000 drugs inventoried, many drugs are perishable (i.e., they have a short shelf-life), and demand for the drugs changes daily. Several factors such as patient condition dynamics and seasonality cause demand to be nonstationary. Moreover, hospitals are structured around clinical departments such as the emergency, intensive care, oncology, catheterization laboratory, operating rooms, nursing units, and inpatient beds, which must have on-hand pharmaceutical products and medical supplies to support patient care. Â
We can develop various optimization and predictive models and algorithms, including but not limited to multi-echelon, multi-supplier inventory optimization models, continuous-time Markov chain models, and demand forecasting algorithms to manage such healthcare inventories more effectively and efficiently. It should be noted that demand, which originates at the patient level, is a defining factor in these inventory optimization models.
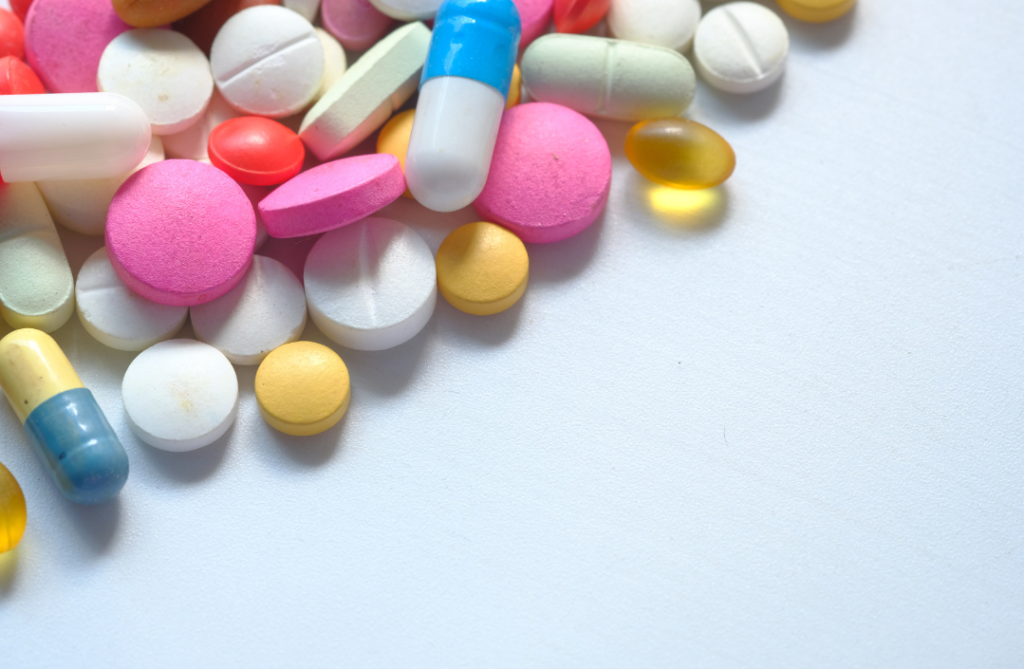
3.2. Operating Room Inventory Optimization
Medical centers such as hospitals struggle to maintain appropriate inventories of operating room supplies. Moreover, nursing units and other clinical departments may frequently run out of critical items used for patient care while overstocked with other surgical supplies. The hospitals’ difficulties in maintaining sufficient operating room inventory levels can cause the following problems:
- Repeated interruptions and delays in surgical schedules.
- Due to a lack of confidence in stocking levels, physicians and staff accumulate stockpiles of operating room supplies to ensure resources would be available when needed.
- Some operating room inventory expires due to overstocking, resulting in considerable financial losses.
We can use optimization techniques to help hospitals fix these issues by:
- Developing a comprehensive long-term inventory control plan.
- Aligning inventory level guidelines with usage and surgical scheduling.
- Eliminating costly overstocks from the operating rooms and other departments such as cardiac catheterization laboratories, radiology, and other vital ancillary areas.
- Identifying wasteful supply chain spending.
Â
Applying operating room inventory optimization to an academic medical center with general and specialty surgery programs has led to a $1.9 million margin improvement and the elimination of unnecessary overstock of more than $1 million.
3.3. Vaccine Supply Chain Optimization
A vaccine supply chain is required to efficiently deliver vaccines from production to the people who need them. A vaccine supply chain has much in common with a commercial supply chain. However, it has several specific characteristics such as perishability, the nonlinearity of health benefits of vaccination, and the non-profitability of vaccination programs for the government as a buyer, which differentiate them from commercial supply chains.
Due to the rising cost of vaccines and the greater storage capacity required at every level of the related cold chain, World Health Organization (WHO) focuses on lower stock levels, reducing wastage, and accurately forecasting vaccine requirements. Therefore, we can use optimization techniques to provide optimal solutions in these three aspects. More specifically, in our vaccine supply chain optimization problems, we should focus on vaccine sourcing from different supply markets, vaccine demand forecasting, vaccine shortage, and vaccine cold chains.
3.4. Blood Supply Chain Optimization
Blood is needed for several treatments, including organ transplants, cancer and anemia treatments, and major surgeries such as open-heart surgery. The demand for blood and blood products is satisfied by the donation of eligible individuals. Although 38% of the population in the U.S. is eligible for donation, only 3% of the population donate blood in a year. The combination of rising demand and shrinking donor pool forces blood donation organizations to be more efficient with their available donors.
A blood supply chain is different from a typical supply chain in three aspects: (1) The number of donations and blood supply change over time. For example, there are seasonal shortages because of low blood collections during the winter and summer months. (2) The whole blood and blood products have short life spans. Therefore, there must be a specific time between two consecutive donor donations to replace the donated blood. (3) To extract platelets, whole blood must be processed within six hours of donation.
The main optimization applications in blood supply chain management include demand forecasting, inventory planning, network design, and vehicle routing. For example, we can consider the following optimization problems in blood supply chains: (1) matching supply with demand via donation tailoring, (2) scheduling collection operations, and (3) inventory management with a focus on the perishability of blood products. In the third problem, we can use the perishable inventory models, considering the average inventory level, the average age of transfused blood, and the average number of shortages and wastage. Our inventory models can focus on the following aspects of the problem: target inventory levels and order policies, issuing policies (FIFO and LIFO), inventory allocation, and distribution policies.
4. Optimization in Medicine
4.1. Diet Optimization
Diet optimization is increasingly used in the field of public health nutrition. We can use diet optimization to identify the optimal combination of foods to answer a given question under a set of predefined constraints such as imposed nutrient recommendations, a total diet cost, total energy content, and an environmental target. For instance, we can use diet optimization to answer the following questions:
- Is it feasible to achieve all the nutritional recommendations (i.e., nutritional adequacy) with the current food supply?
- What is the minimum cost of a nutritionally adequate diet?
- Which optimal food combinations would be required to reach nutritional adequacy while staying as close as possible to the current diet?
- What is the optimal combination of foods to reduce environmental impact while fulfilling nutrient recommendations and departing the least from an existing diet?
We can use different mathematical modeling techniques to implement a diet optimization tool based on the unique features of the given case and its available data.
4.2. Radiation Treatment Optimization
Patients with a tumor should receive radiation therapy. During radiation therapy, an external beam is passed through the body, harming both cancerous and healthy tissue. In practice, many beams are used simultaneously. For example, brain cancer needs two different beams. We can use optimization techniques to minimize damage to healthy tissue while delivering the required dose to cancer tissue. As an example, we can define exposure times for beams as decision variables of our optimization model. In this case, our optimization model aims to select beam durations to generate the best dose distribution. We should include the treatment requirements such as the delivered dose being sufficient to kill the malignant cells and the aggregate dose not exceeding established tolerance levels in our optimization model as constraints.
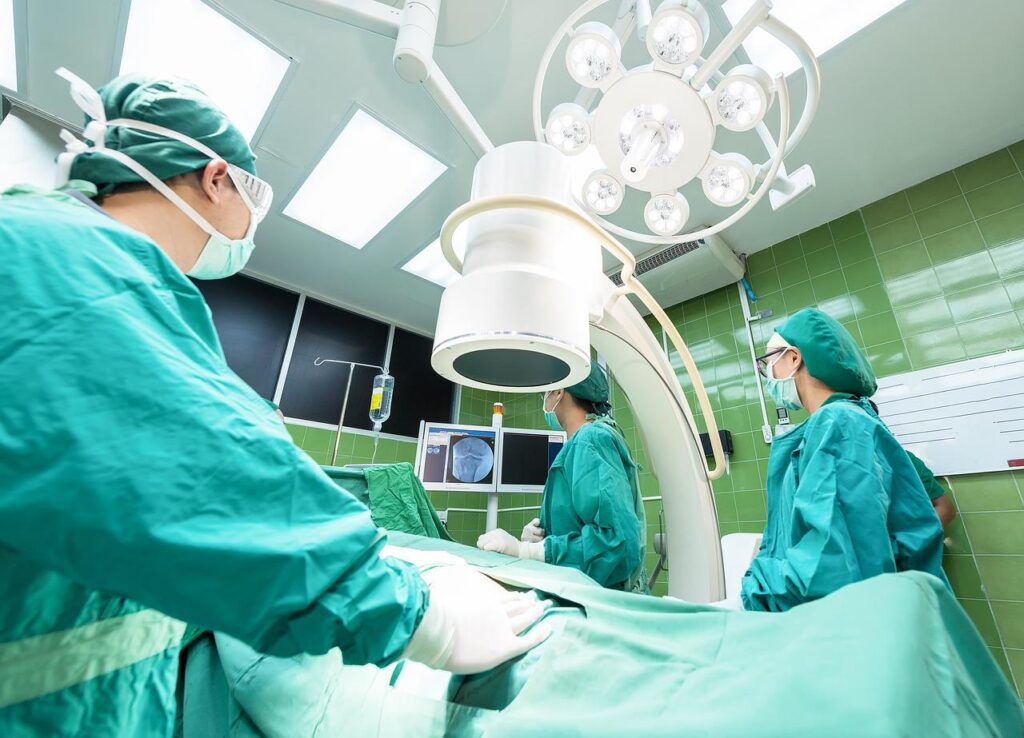