In an era where data is often called the new oil, organizations across industries are constantly seeking ways to extract valuable insights from the vast amounts of information at their disposal. Enter IBM SPSS – a comprehensive suite of statistical and predictive analytics tools that has been at the forefront of data analysis for over half a century. In this blog post, we’ll take an in-depth look at the SPSS suite, its components, features, real-world applications, and its place in the broader landscape of data science and artificial intelligence.
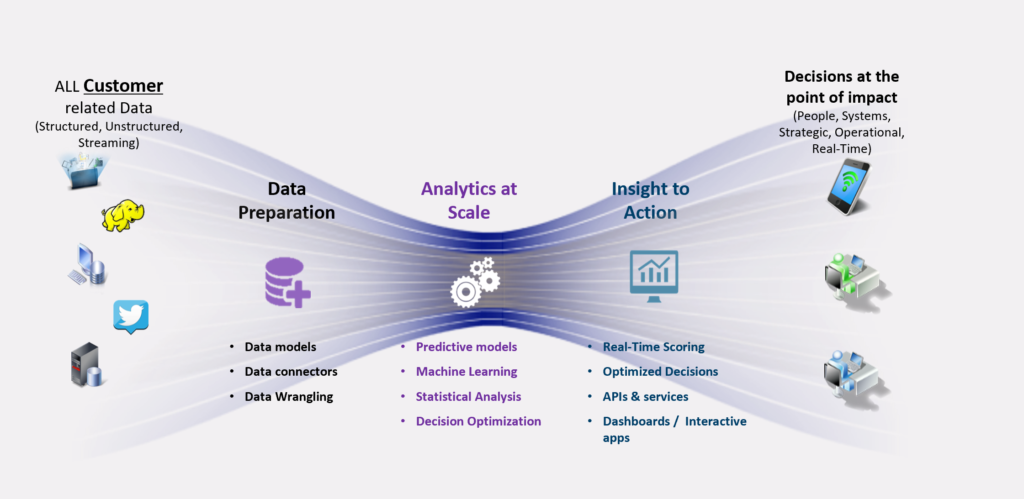
The Evolution of SPSS
SPSS, which originally stood for Statistical Package for the Social Sciences, was first released in 1968. What started as a tool primarily for social scientists has evolved into a powerful analytics platform used across various industries. Today, the SPSS suite consists of two main products: SPSS Statistics and SPSS Modeler, each serving distinct but complementary purposes in the data analysis ecosystem.
SPSS Statistics: The Statistical Powerhouse
SPSS Statistics is widely recognized as the world’s leading statistical software. It enables users to dig deeper into their data, making it a far more effective tool than spreadsheets or standard multi-dimensional tools for analytics. Let’s explore its key features and strengths:
SPSS Statistics offers an intuitive interface that makes it accessible to both beginners and advanced users. It allows for point-and-click operation while also supporting syntax for more complex analyses. The software provides a wide range of statistical procedures, from basic descriptive statistics to advanced techniques such as linear and nonlinear models, cluster analysis, and factor analysis.
One of the strengths of SPSS Statistics is its ability to excel at data cleaning, transformation, and restructuring, saving analysts valuable time in the data preparation phase. The software is particularly strong in hypothesis testing, allowing researchers to validate or disprove assumptions quickly and accurately.
When it comes to presenting findings, SPSS Statistics offers robust capabilities for creating charts, graphs, and tables to communicate results effectively. Users can also leverage R and Python within SPSS Statistics, combining the software’s ease of use with the flexibility of these popular programming languages.
For those looking to expand the software’s capabilities, the SPSS Extension Hub provides access to over 100 extensions, allowing users to add specialized tools and functions to their workflow. SPSS Statistics is particularly popular in academia, market research, healthcare, and government agencies where rigorous statistical analysis is crucial for decision-making.
SPSS Modeler: Visual Data Science and Machine Learning
While SPSS Statistics focuses on traditional statistical analysis, SPSS Modeler is designed for visual data science and machine learning. It aims to help enterprises accelerate time-to-value by speeding up operational tasks for data scientists.
SPSS Modeler offers a drag-and-drop interface that doesn’t require programming skills, making it accessible to citizen data scientists and business analysts. The software includes automated modeling capabilities that can test multiple algorithms and create ensemble models, saving time and improving model accuracy.
In terms of analytical capabilities, SPSS Modeler supports a wide range of techniques, including classification, segmentation, association analysis, and time series forecasting. The software can also extract concepts and patterns from unstructured text data, enhancing models with insights from sources like social media, customer feedback, and open-ended survey responses.
For organizations dealing with large datasets, SPSS Modeler can work with big data platforms like Hadoop and Apache Spark for scalable analytics. It also incorporates geospatial analytics, allowing users to include geographic data in their analyses and improve model accuracy for location-dependent problems.
Like SPSS Statistics, Modeler allows users to incorporate R, Python, and Spark algorithms into their workflows, providing flexibility and extensibility. SPSS Modeler is particularly useful for tasks such as customer segmentation, fraud detection, risk assessment, and predictive maintenance across industries like finance, retail, manufacturing, and telecommunications.
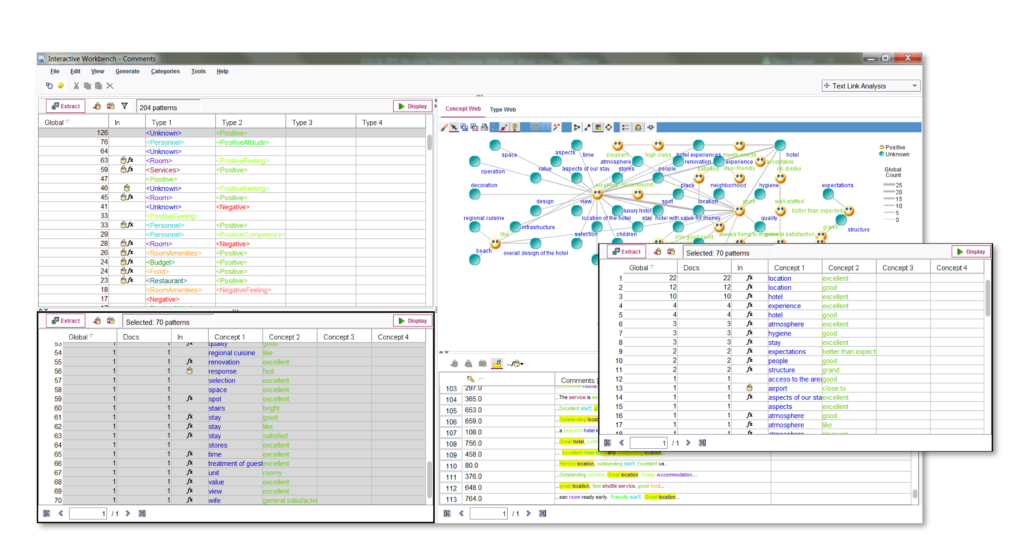
The SPSS Ecosystem: Deployment and Scalability
One of the strengths of the SPSS suite is its flexibility in deployment and scalability. SPSS can be deployed on-premises, in the cloud, or in hybrid environments, giving organizations the flexibility to choose the best setup for their needs. The SPSS Collaboration and Deployment Services component allows for enterprise-wide sharing and management of analytical assets, promoting collaboration and standardization across teams.
For organizations dealing with large-scale analyses, SPSS can push certain operations to the database level, reducing data movement and improving performance. Models developed in SPSS can also be deployed for real-time scoring, enabling organizations to make instant decisions based on incoming data.
Furthermore, SPSS is now closely integrated with IBM Watson Studio, providing a comprehensive environment for data science and machine learning that combines the strengths of traditional statistical analysis with cutting-edge AI techniques.
Need SPSS Training? Enroll for
Real-World Impact: SPSS in Action
The power of SPSS is evident in its real-world applications across various industries. A leading financial services company reduced the time to build and deploy real-time models for predicting client life events from 2 months to just 14 days using SPSS Modeler. This dramatic improvement allowed them to offer more timely and relevant products to their clients, enhancing customer satisfaction and driving business growth.
In the transportation sector, a truck manufacturer implemented predictive maintenance using SPSS Modeler, reducing diagnostic time by up to 50% and repair time by more than 20%. By predicting component failures before they occur, the company was able to minimize unplanned downtime, saving costs and improving customer satisfaction.
The education sector has also benefited from SPSS. The Keller Graduate School of Management at DeVry University used SPSS Statistics to identify at-risk students, resulting in a 2% increase in persistence rates and a 2% growth in student satisfaction. By combining data from multiple sources and extracting useful insights, the school was able to target effective intervention activities and help students reach their full potential.
Law enforcement agencies have found value in SPSS as well. Police Charleroi in Belgium uses SPSS Analytics to optimize their operations and ensure they meet targeted response times. By performing statistical analyses on incident data, they produce heat-maps and dashboards to help deploy resources in the right places at the right times, improving crime prevention efforts.
In the retail sector, Grupo Boticário, a leading Brazilian cosmetics company, uses SPSS Modeler to predict consumer demand for their products. This data-driven approach has helped them optimize their inventory management and marketing strategies, leading to improved sales and customer satisfaction.
Public transportation has also seen improvements through SPSS. Dallas Area Rapid Transit (DART) implemented SPSS Modeler to aggregate data from five different sources, gaining better insight into daily operations for rail ridership and operation management. This has allowed them to better understand factors affecting light rail ridership and make more informed decisions about train scheduling and maintenance.
Even large-scale event management has benefited from SPSS. Copenhagen Business School used SPSS Modeler along with other IBM technologies to help manage the Roskilde Festival, one of Europe’s largest music festivals. The analytics solution provides fast insights into crowd movements and sales data, supporting sustainability efforts and optimizing safety, catering, energy, water, and waste management for the 130,000 attendees.
These case studies demonstrate the versatility and power of SPSS across different sectors, showcasing its ability to drive tangible business outcomes and societal benefits.
Â
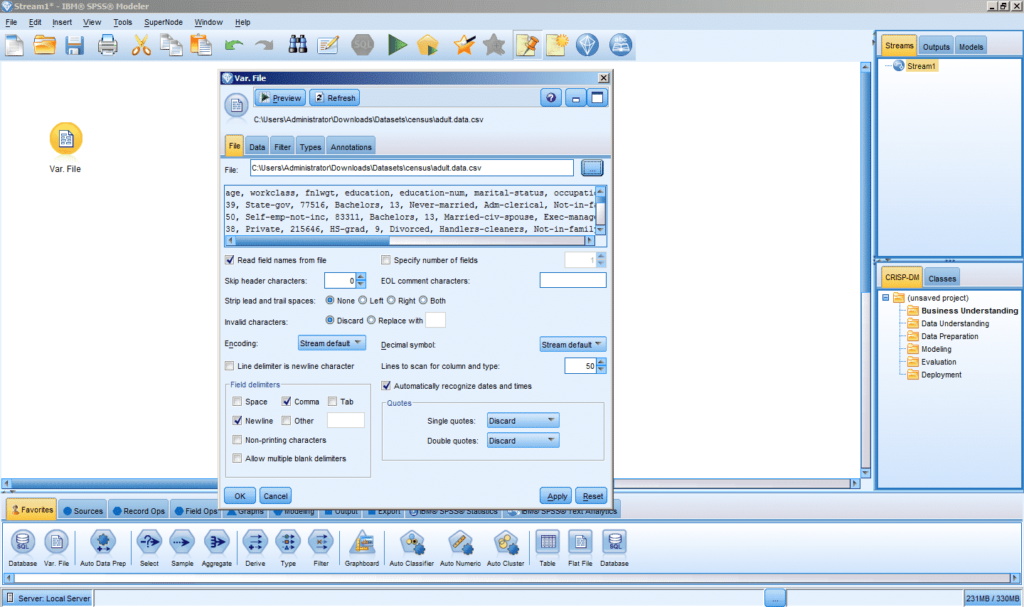
SPSS and the Future of Data Science
As data science and artificial intelligence continue to evolve, SPSS is adapting to meet the changing needs of organizations. The integration with IBM Watson Studio positions SPSS as part of a broader AI and machine learning ecosystem, allowing users to leverage advanced AI capabilities alongside traditional statistical techniques.
SPSS is incorporating more automated machine learning capabilities, making it easier for non-expert users to build and deploy sophisticated models. With flexible deployment options, SPSS is well-positioned to support organizations as they migrate to cloud and hybrid environments.
To address the challenges of big data and the Internet of Things (IoT), SPSS continues to enhance its capabilities for handling large data streams, enabling real-time analytics at scale. As interpretability becomes increasingly important in AI and machine learning, SPSS is focusing on providing tools for explainable AI, helping users understand and communicate the rationale behind model predictions.
Conclusion
IBM SPSS remains a cornerstone in the world of data analysis and predictive analytics. Its combination of user-friendly interfaces, powerful statistical and machine learning capabilities, and flexibility in deployment make it a valuable tool for organizations of all sizes across various industries.
As we move further into the age of big data and AI, tools like SPSS will play an increasingly crucial role in helping organizations turn raw data into actionable insights. Whether you’re a researcher conducting complex statistical analyses, a data scientist building predictive models, or a business analyst seeking to understand customer behavior, SPSS offers a robust and adaptable solution to meet your needs.
The future of data-driven decision making is here, and IBM SPSS is at the forefront, empowering organizations to unlock the full potential of their data and drive innovation in an increasingly competitive landscape.
Want to Buy SPSS? Visit
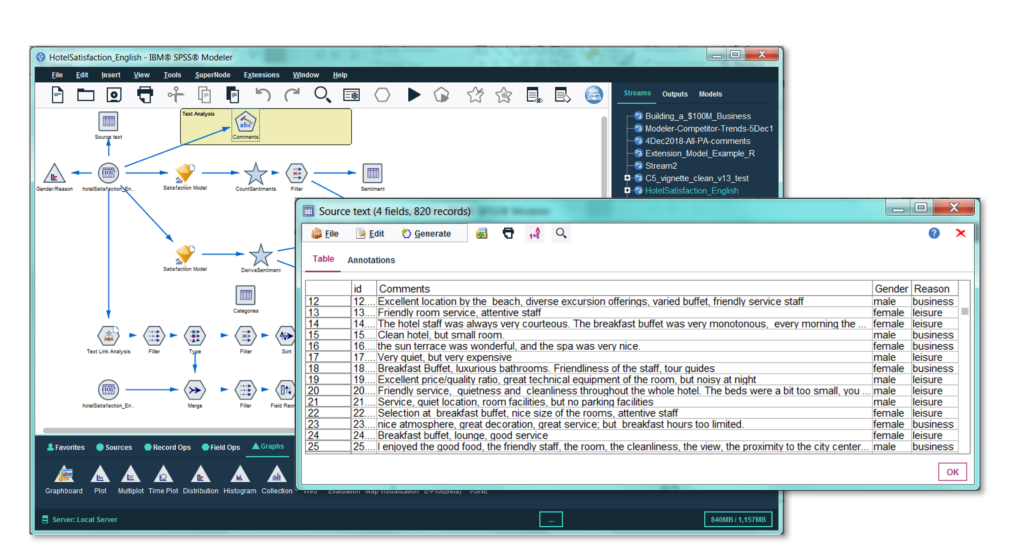
How Cresco Can Help
For organizations looking to harness the full potential of IBM SPSS and transform their data analysis capabilities, Cresco International stands out as a valuable partner. As an IBM Business Partner, Cresco International specializes in implementing and optimizing IBM SPSS solutions across various industries. Their team of experienced consultants brings deep expertise in both the technical aspects of SPSS and its practical applications in business contexts. Whether you’re just starting your journey with SPSS or looking to enhance your existing analytics infrastructure, Cresco International can provide tailored guidance and support. They offer services ranging from initial assessment and strategy development to full-scale implementation, training, and ongoing support. With their help, organizations can navigate the complexities of data science and machine learning, ensuring that they extract maximum value from their SPSS investment. Cresco International’s track record includes successful SPSS implementations for clients in various sectors demonstrating their ability to adapt SPSS solutions to diverse business needs. By partnering with Cresco International, businesses can accelerate their analytics maturity, drive data-driven decision making, and unlock new opportunities for growth and innovation. As the data landscape continues to evolve, having a knowledgeable partner like Cresco International can be invaluable in staying ahead of the curve and fully leveraging the power of IBM SPSS.