Competition within the insurance industry is on the rise. To stay in the market, insurance companies face a lot of challenges including but not limited to reducing costs while maintaining, if not improving, the quality of provided services and offers. Advanced analytics such as optimization solutions is one of the tools that can help insurance managers and decision-makers reach their goals. In what follows, we will explain how decision-support optimization solutions powered by Artificial Intelligence (AI), Machine Learning (ML), and Optimization models and algorithms can help insurance companies.
1. Fraud Detection
According to studies, 1 in every 10 insurance claims turns out to be fraudulent, and fraud makes up 5-10% of claims costs for insurers in the United States and Canada. As a result, $80 billion is lost annually from fraudulent claims in the United States alone. However, AI and ML can help insurers identify and prevent potential fraud before it happens or pursue corrective measures retroactively. For example, AI and ML can analyze the insured’s past behavior, frequency of claims, credit score, etc., and identify mismatches between the insured party, third parties involved in the claim (e.g., repair shops), and even the insured party’s social media medial accounts and online activity. Moreover, AI-based and ML-based optimization solutions can improve agents’ policy work accuracy and consistency by reducing the amount of guesswork entailed in decisions. For example, the system can guide adjusters with limited experience to avoid overcharging a client for a policy or undercompensating for a claim.
We can develop a predictive modeling and decision-support optimization solution for early claim resolution using AI and ML. This optimization solution can reduce the claim costs between %3 to %10 by automating low-risk claims and identifying higher-risk claims shortly after the report. Indeed, it can send high-risk claims to senior adjusters for intervention and cost containment while identifying low-risk claims for straight-through Processing. Â To that end, the tool: 1) leverages a wide range of raw, unstructured claims data to predict and plan for volatile and high-cost claims by extracting information from adjuster notes shortly after the claim is reported; and 2) visualizes data dynamically to quickly identify insurance claims anomalies leading to the detection of problematic claims early.
This optimization solution will provide:
- An interactive claims summary dashboard that is refreshed daily where managers are provided with a dashboard view with aggregated KPIs vital to their business. Unlike a static report, the interactive dashboard allows drill-down into the source systems for further analysis.
- A summary of the claims trends by the line of business, industry or cause of loss, and more.
- Key metrics such as Average Number of Days to Close, Reserve Deviation, etc.
- Quick insights on the priorities of the fraud referrals based on priority scores calculated by a predictive model.
- Additional information such as the source of the referral, a summary of the loss, and best adjustor recommendations for a specific claim.
- A comprehensive view of the risk factors in the claim region and a Fraud Heat Map
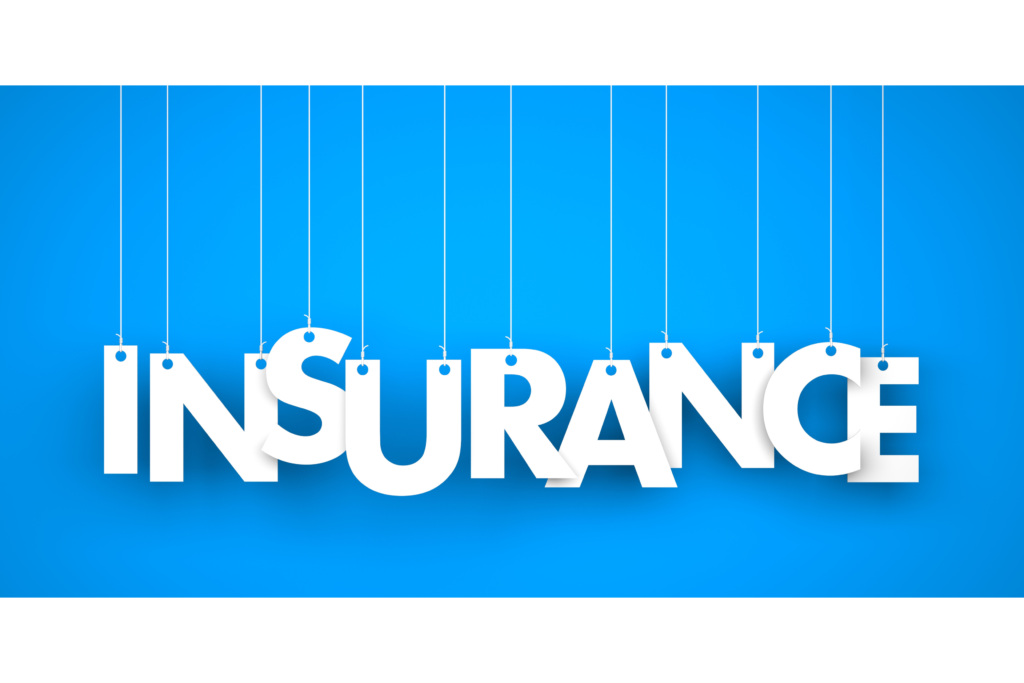
2. Underwriting Automation
Insurance underwriting is a complex process involving a series of decisions that are both complex and high-impact. Unfortunately, many underwriters get bogged down trying to search, access, aggregate, and visualize the data they need to make those decisions. As a result, they spend more time on low-level administrative tasks rather than on actions that can lower risk and increase profitability for the organization. Indeed, underwriters often spend 50 percent or more of their time gathering information. However, underwriters can be empowered by AI and ML. The interactive optimization solutions powered by AI and ML will: 1) help underwriters handle substantially larger books of business with more precision and control; 2) use data throughout the underwriting process to inform underwriter decisions in prioritization of prospects, validation of exposures, policy structuring, and pricing; and 3) adopt risk models that incorporate ever-expanding views of risk characteristics, tailored by line, segment, and emerging-loss trends.
We can develop an optimization solution with an intuitive front-end user interface that uses AI and ML to deliver a quote and bind a policy in minutes rather than days. Although the platform will still rely on agents’ input, we will equip it with a new straight-through processing risk-assessment engine that minimizes manual effort. Our optimization solution can increase the efficiency of the underwriting process by dramatically simplifying and improving the agents’ experience through:
- Diverting low-risk accounts to straight-through Processing. Up to 95% of policies may undergo straight-through Processing with no underwriter involvement.
- Routing only complex risks to underwriters for review.
- Decreasing manual inputs by up to 90%.
- Conducting granular segmentations of risk and applying advanced modeling techniques that take the regulatory landscape into account. These models identify the risk characteristics that help to improve pricing and reduce losses.
- Reducing turnaround times for decisions.
Our tool applies the following 3-step process:
- Collecting relevant client information, including credit history, medical history, and driving record.
- Performing rigorous risk assessments by analyzing data to devise a risk score for the insured party.
- Applying predefined underwriting guidelines to accept or decline the application for the policy, and if accepted, calculating the client’s premium based on the obtained risk score.
This tool will:
- Aggregate data from multiple sources into a single repository for faster access and flexible deployment
- Help underwriters access all their data and insights within a single user interface, along with guidance to help them make faster, more strategic, more profitable submission decisions.
- Save significant time for each underwriter by eliminating data gathering, analysis, and visualization tasks to surface relevant insights throughout an end user’s workflow
- Help underwriting managers route each submission to the most qualified agent for an optimal business outcome, rather than sending it to the first available agent
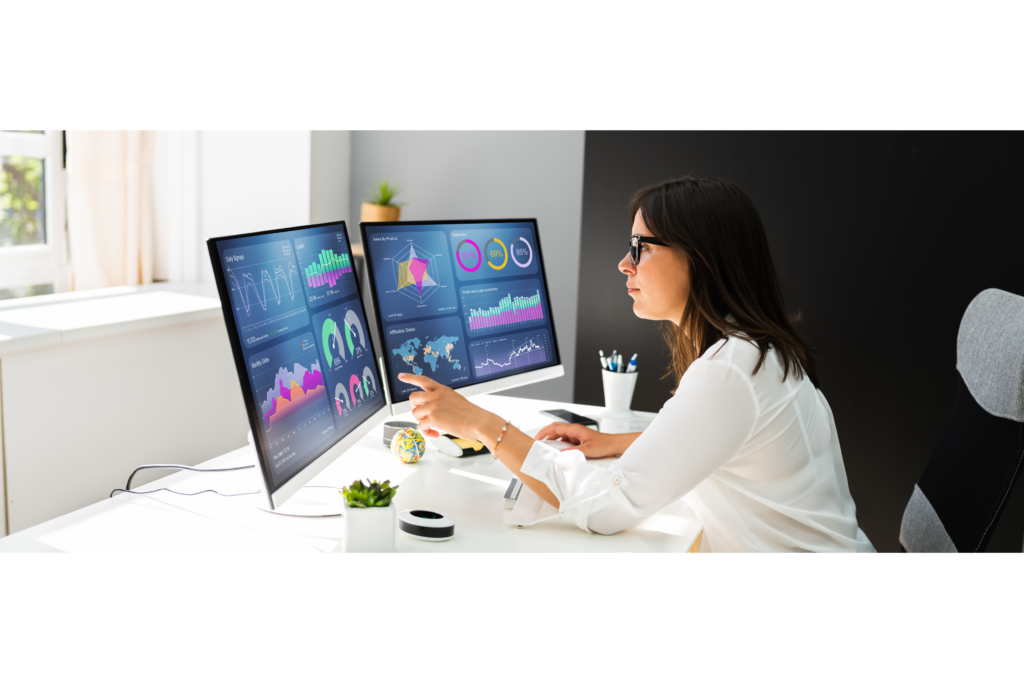
3. Pricing Optimization
Traditional pricing tools cannot always offer fair premiums to applicants because they give too much weight to some factors like age. For example, they offer higher premiums to younger drivers only because of their age, even if they drive very safely. Thus, younger customers are not motivated to drive safely based on the premium, and retention rates are therefore lower. We can develop an advanced pricing tool based on ML models that are fitted to the policyholder’s vehicle and consider a whole range of data about their driving style. Our ML-based pricing tool can be tailored to the insurer’s current pricing structure. More specifically, the premiums can be priced within existing pricing bands as the ML-based pricing tool assesses risk and chooses the optimal price band for each customer.
Our ML-based pricing tool can improve pricing accuracy for customers, giving them fairer premiums than with non-ML-based pricing tools. For example, unlike traditional tools, our ML-based pricing tool can offer lower premiums to low-risk younger drivers by considering other variables that indicate the lower risk. Therefore, the younger drivers are more likely to choose the insurer. This leads to increased customer satisfaction, a greater sense of loyalty to the insurer, and higher retention rates. Our ML-based pricing tool can also improve risk sensitivity by finding claim data patterns and linking them to customer or policy characteristics. Our tool’s enhanced risk sensitivity will:
- Charge new customers appropriately according to these improved predictions.
- Lead to insurers’ better profitability and a lower loss ratio.
- Allow insurers to adjust their prices dynamically to meet specific financial targets.
4. Identifying Customers at Risk of Cancellation
We can use ML and AI to develop a tool that can identify the customers who are likely to cancel or lower coverage. Our tool uses advanced data insights to identify customers who may be unhappy with their coverage. Having this knowledge in hand will put insurers ahead of the game and allow them to reach out and provide personalized attention to alleviate potential issues. Without such a predictive tool, insurers could miss credible warning signs and lose valuable time that could be used to remedy any issues.
5. Claims Processing
The average time required for claims settlement is one of the critical factors that determine the overall efficiency of an insurer. Claims processing includes multiple time-consuming steps and numerous issues like manual or inconsistent Processing, varying data formats, and changing regulations may happen while performing these steps.
We can develop an efficient claims processing tool that can lead to faster claims settlement. Our tool’s strong big data analytics capabilities in Processing and analyzing massive datasets can make various aspects of a claim processing faster, simplifying the overall process of claim settlements. Also, with ML and AI, our tool can analyze historical data to determine events, which could affect the outcome of claims. This can streamline the entire claims process and help mitigate risks.
This tool will:
- Kickstart the claims processing by providing claims insights quickly with pre-built templates for dashboards, data mapping, and reports, as well as predefined data models
- Present a 360-degree view of the claims by bringing together internal and external data sources
- Incorporate predefined data input templates to integrate data from multiple internal and external sources rapidly
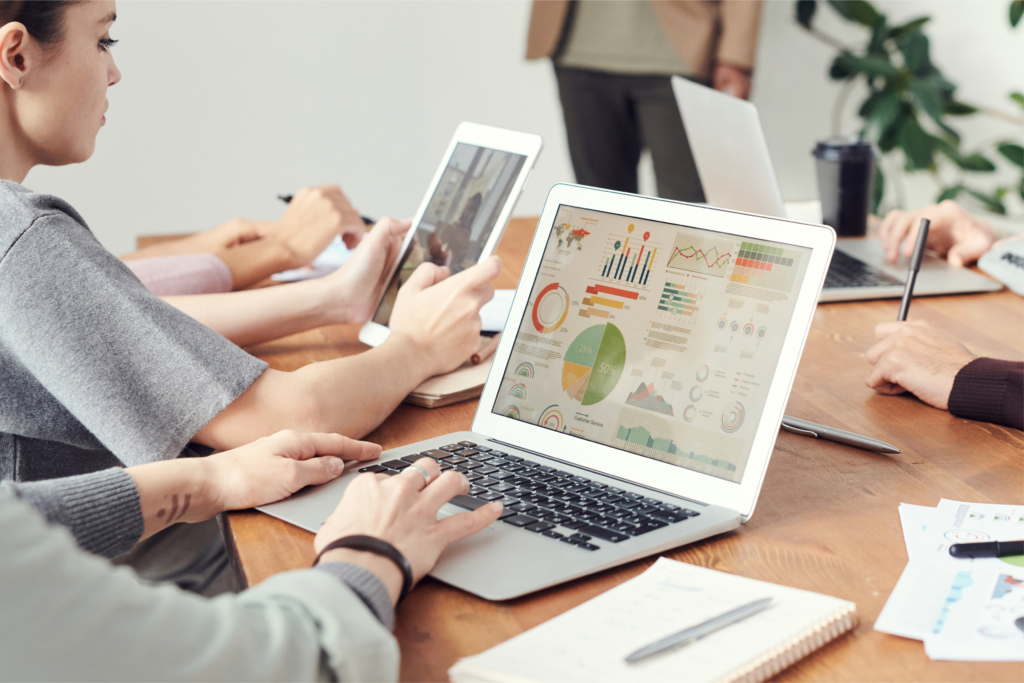
6. Real-time Schedule Adjustment Optimization
In call centers, agents’ schedules are created several days or weeks before the time that agents report to work. After creating schedules, call center managers may receive additional information that can affect forecasted workload and resource availability. We can develop optimization solutions for real-time schedule adjustments to make intra-day resource adjustment decisions that take into account updated call forecasts, updated agent requirements, existing agent schedules, agents’ schedule flexibility, and associated incremental labor costs. Adjustments are made on an intra-day basis to agents’ schedules, and there is typically a limited set of feasible adjustments that can be made due to human resource policies and practices. The optimal real-time schedule adjustments provided by our optimization solutions can decrease operating costs and increase service quality.
7. Skill-based Contact Routing and Staffing Optimization
Although the diversity of channels (e.g., calls, chats, faxes, emails, callbacks) in a contact center can help smooth workload, the operational challenges faced by the contact center managers are also more complicated with such a system that has multi-skill agents and customers that arrive from various channels with heterogeneous rates and waiting behaviors. One of the fundamental challenges contact center managers faces is how to schedule agents to meet the quality of service targets at minimum costs. In a contact center, almost 60-80% of the operating budget is comprised of the cost of agents.
We can develop routing and staffing optimization solutions for multi-skill contact centers. This tool determines the optimal assignment of calls, chats, faxes, emails, and callbacks to agents while at the same time determining the optimal agents’ work schedules. Indeed, our tool can deploy the right number of agents with the right skills to the right schedules (shifts) to meet the uncertain, time-varying service demands.
8. Call Center Workforce Optimization for Outbound or Hybrid Call Centers
Many insurance companies require the distinctive direction of their call center workflow. They may handle either only inbound or outbound calls or might deal with a combination of both inbound or outbound calls. We can develop workforce optimization tools for outbound call centers or hybrid call centers too. These Optimization tools can optimize the call center’s goals from different points of view. For example, an optimization tool can assign both inbound and outbound calls to agents, while another Optimization tool may assign different agents to handle inbound and outbound calls separately.
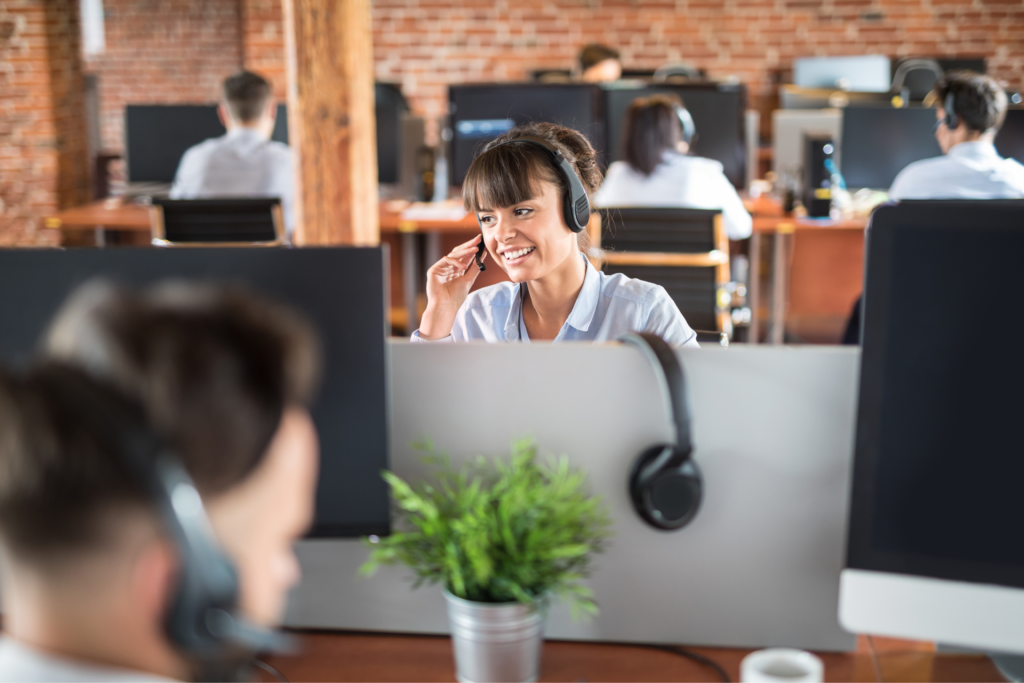
9. Optimization of Insurance Portfolios
The liability stream of insurance companies often stretches several years into the future. Therefore, there is always the need to determine a portfolio of bonds or other assets whose cash-flows replicate those of the liability stream. Insurance regulatory authorities require that insurance companies must demonstrate solvency. To achieve this, an insurance company needs to determine a fair market value of its liability by finding a replicating portfolio consisting of default-free bonds.
We can develop an optimization insurance portfolio tool based on stochastic optimization models. This tool can be employed for insurance portfolio optimization in the presence of background risk. The optimal solutions provided by this tool answer the question of whether the new risk is a good addition to the existing portfolio or not.
10. Catastrophe Modeling
We can develop optimization tools based on catastrophe modeling, which is becoming increasingly important to insurance companies as they make decisions on catastrophe coverages, premiums, reinsurance agreements, and the effects of mitigation measures (earthquakes, floods, tornados, etc. are considered as catastrophic events). For example, we can develop an approach that integrates catastrophe modeling with stochastic optimization techniques to support decision-making on coverages of losses, profits, stability, and insurers’ survival. Â It is possible to simulate catastrophes in a region and analyze the impact of different combinations of decision variables on the performance of insurance companies. Â A catastrophe may affect different locations and produce rare and highly correlated losses in space and time. It may ruin many insurers if their risk exposures are not properly diversified among locations. The multidimensional distribution of claims from different locations depends on decision variables such as the insurer’s coverage at different locations, spatial and temporal characteristics of possible catastrophes, and insured values’ vulnerability.
11. Renewal Price Adjustment Optimization
In the insurance industry, an important question arises: how can insurance renewal prices be adjusted? Such an adjustment has two conflicting objectives. On the one hand, insurers are forced to retain existing customers. On the other hand, insurers are also forced to increase revenue. Intuitively, one might assume that revenue increases by offering high renewal prices. However, this might also cause many customers to terminate their contracts. Contrarily, low renewal prices help retain most existing customers but could negatively affect revenue. Therefore, adjusting renewal prices is a non-trivial problem for the insurance industry.
We can develop an optimization tool for renewal price adjustment. In particular, the renewal price adjustment problem is considered as follows: The insurer has a portfolio of customers. Then, when it is time to renew the customer prices, the insurer takes the first client from the portfolio and decides which renewal price to offer him/her, taking into account the company’s current situation (i.e., current revenue retention). Whether the customer accepts the renewal price or not, the insurer’s decision leads the company to a new situation (e.g., if the customer does not accept the renewal price, the insurance company will have one customer less and, hence, lower revenue). Additionally, we can know if the insurer’s decision has been good or bad (e.g., if the insurer’s decision increases the revenue, we can consider that the decision has been good and bad otherwise). Thus, we can take into account the utility of each particular decision. Then, given the new situation of the company, the insurer takes the next customer from the portfolio, makes a decision, and so on until the insurer makes a decision for each of the customers in the portfolio. In this way, the problem can be seen as a succession of situations (states), decisions (actions), and utilities (rewards). Therefore, we can model this problem as a Markov decision process, which provides two benefits: it takes into account the long-term effects of each decision and takes into account the expected value of each decision. This tool optimizes each particular customer’s decision considering the sequential process involved and the optimization criteria selected (e.g., maximization of the revenue).
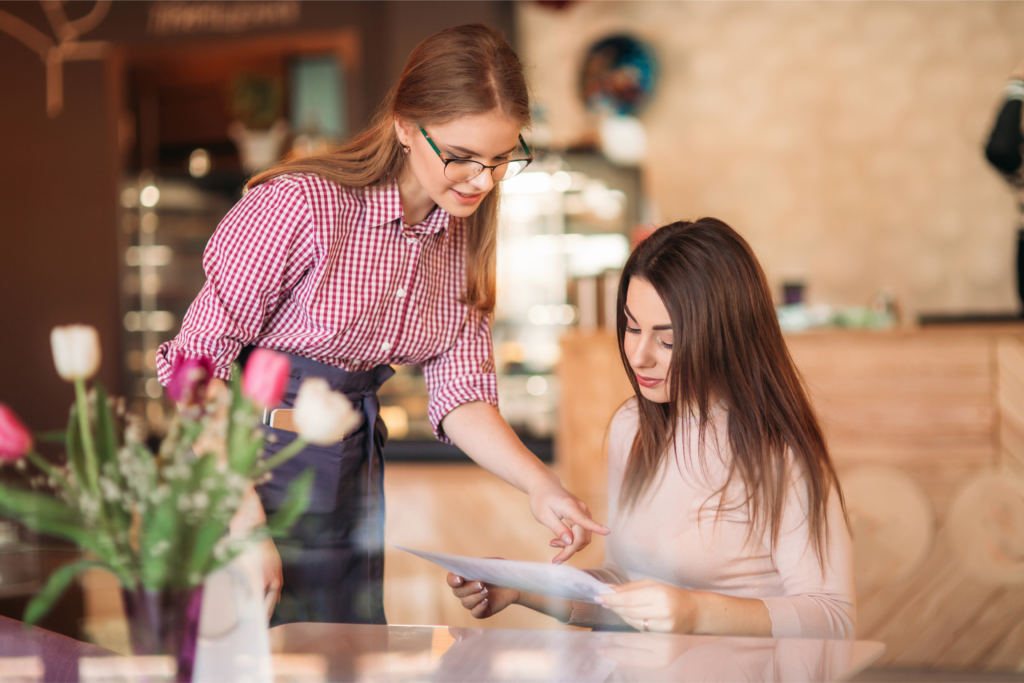
12. Rating Factor Optimization
It is challenging to manage rates and ensure consistency during rate changes. So, insurers should try to minimize rate disruption. Sometimes they use trial-and-error, which is highly time-consuming. In many cases, existing rates are rates in a table, and no factor-based relationships among them are considered. To change the final price of the policy, insurers need to change the value of several factor parameters such as driver’s age, driver’s gender, and car’s age. But the change in each factor influences more than one risk profile.
We can develop a rating factor optimization tool to optimize the factor values directly rather than the final price of a policy. In addition to the insurer’s business constraints, we can consider other constraints such as monotonicity of rate factors, the maximum change from the current rate by each factor (e.g., not more than +/-2% relative to the current value).