Introduction
In the dynamic landscape of modern workplaces, the fusion of Operations Research (OR), Data Science, and Artificial Intelligence (AI) is revolutionizing the approach to employee well-being. This blog delves into the transformative power of these technologies, exploring their application in optimizing various aspects of the workplace. From workforce scheduling to health analytics and AI-driven engagement tools, the strategic integration of OR, Data Science, and AI emerges as a pivotal force in shaping environments that prioritize employee satisfaction, productivity, and holistic well-being.
As organizations navigate the complexities of the contemporary workforce, the intelligent use of OR, Data Science, and AI promises not only operational efficiency but also a genuine commitment to nurturing the well-being of employees. From personalized talent management strategies to innovative workplace designs, these technologies stand at the forefront of creating work environments that are not only adaptive but also deeply considerate of the diverse needs and aspirations of the workforce.
1. Operations Research (OR) Use Cases for Employee Well-Being
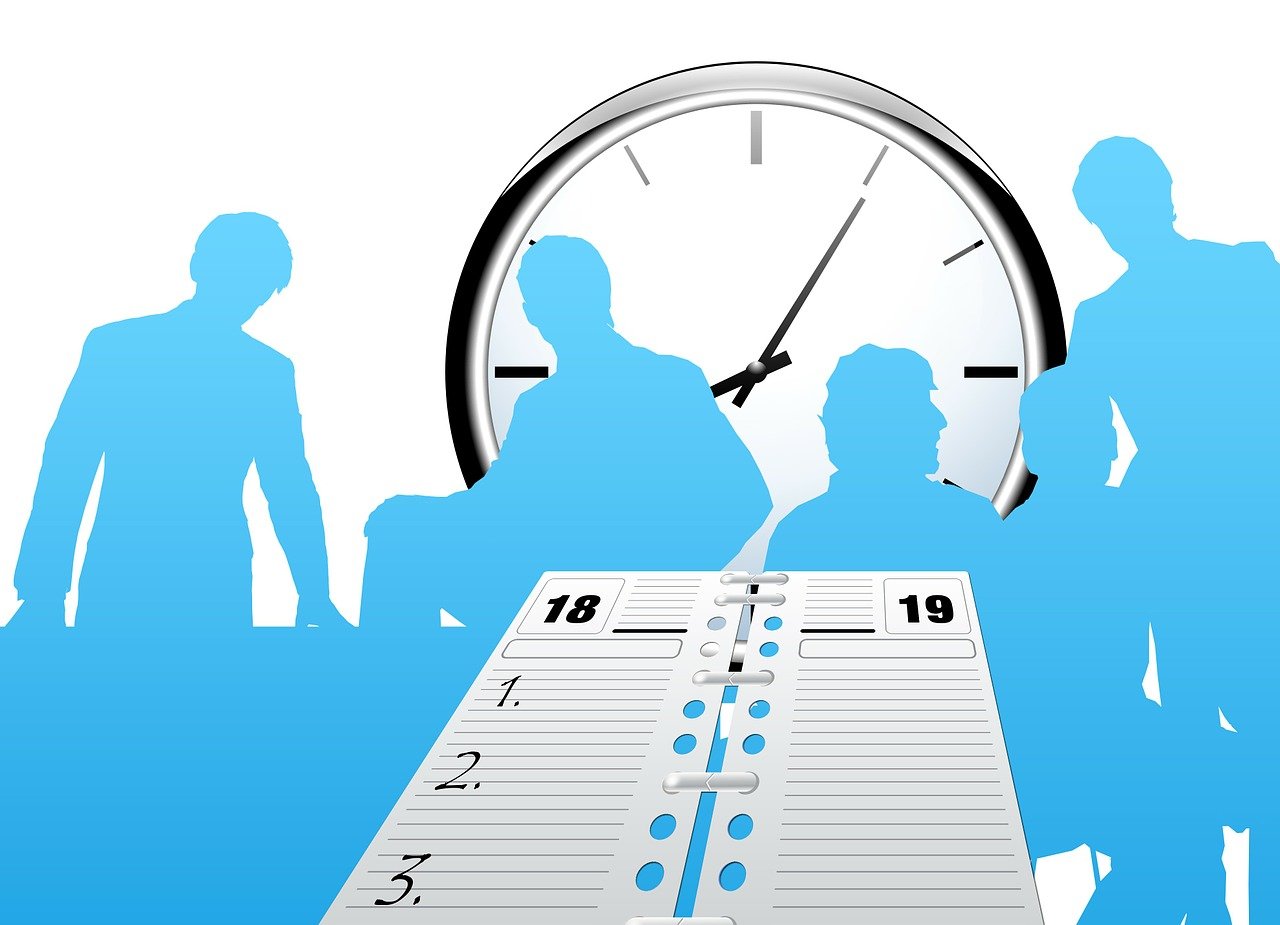
1.1. Workforce Scheduling Optimization
In the realm of workforce scheduling, Operations Research (OR) stands as a powerful tool that extends beyond mere time management. Workforce scheduling optimization provides efficient and balanced work
schedules, taking into account employee preferences, skills, and workload. It ensures fair distribution of tasks and workload, preventing burnout among employees. Moreover, it can help ensure that departments are neither understaffed nor overstaffed, improving productivity and employee satisfaction.
OR algorithms not only align preferences, skills, and workload but also take into account external factors such as peak productivity hours and employee preferences for work-life balance. This optimization process is dynamic, adapting in real-time to changing conditions, ensuring not only operational efficiency but also the adaptability needed to meet evolving employee needs. The optimization process involves linear programming and stochastic modeling to dynamically adapt to changing conditions, ensuring operational efficiency and adaptability.
Real-time adjustments are facilitated by machine learning algorithms that learn from historical data, making the scheduling process not just efficient but also highly responsive to evolving employee needs. Through this approach, organizations can foster a work environment that is not only productive but also considerate of the well-being of their workforce.
1.2. Facility Layout Optimization
Facility Layout Optimization is about Optimizing the physical layout of workspaces to enhance collaboration, communication, and ease of access. The optimization of physical workspaces is a multifaceted challenge, and OR addresses it by going beyond mere efficiency. It considers social dynamics, team structures, and communication patterns to design layouts that promote collaboration and innovation. Importantly, OR takes into account psychological aspects of the workplace, creating environments that positively impact employee mental well-being and foster a sense of community. This holistic approach to facility layout optimization contributes not only to productivity but also to the overall satisfaction and engagement of employees.
Facility layout optimization with OR involves complex spatial analysis and graph theory. Social network analysis is applied to understand team structures and communication patterns, influencing the
arrangement of physical workspaces. Moreover, OR utilizes cognitive ergonomics principles to design layouts that positively impact employee mental well-being. This includes considerations for lighting, noise reduction, and ergonomic furniture. Simulation models, based on queuing theory, are often employed to test and refine layouts before implementation.
1.3. Talent Acquisition and Retention Optimization
OR streamlines the talent acquisition process by employing mathematical models and algorithms that match candidates with job positions based on a nuanced understanding of both job requirements and candidate attributes. This data-driven approach ensures a more accurate and efficient hiring process. Furthermore, it extends its capabilities to retention by identifying patterns in employee satisfaction, suggesting personalized strategies to retain key talent, and contributing to a stable and motivated workforce. Retention optimization involves the application of statistical models to identify patterns in employee satisfaction, using predictive analytics to forecast potential attrition. The marriage of technology and human insight enhances the organization’s ability to attract, develop, and retain top talent.
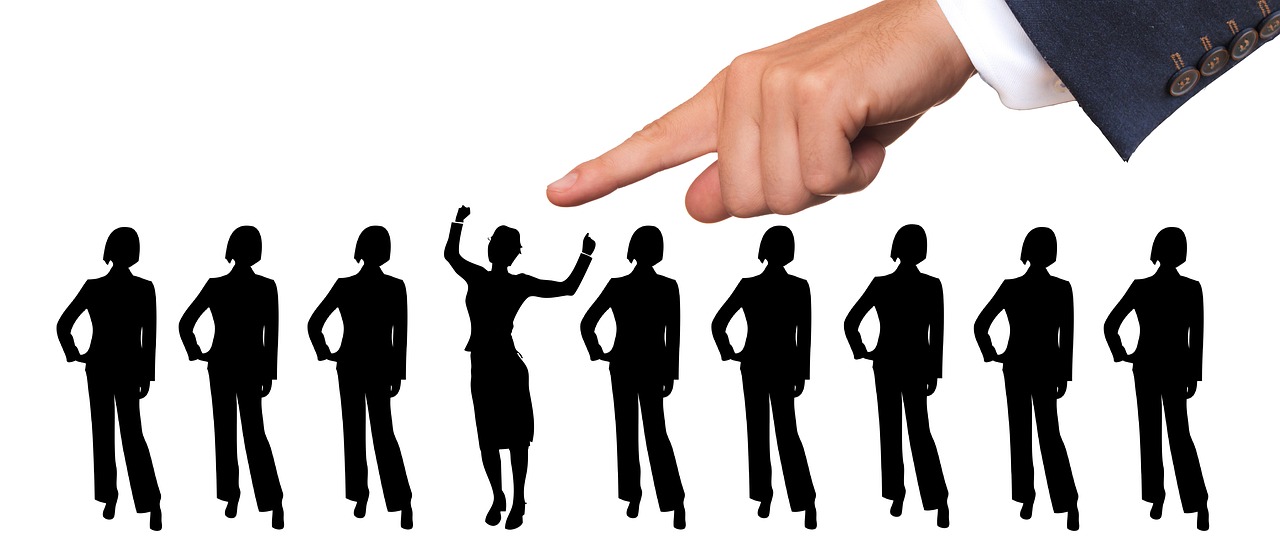
1.4. Resource Allocation Optimization
Efficient resource allocation is crucial for employee satisfaction and long-term engagement. OR takes a comprehensive approach by not only considering skills and motivation but also factoring in career development goals and aspirations. This results in a strategic alignment of tasks with individual strengths, fostering a sense of purpose and contributing to long-term job satisfaction. The data-driven insights provided by OR enable organizations to create an environment where employees feel valued and their professional growth is prioritized.
Â
Resource allocation optimization with OR involves the application of multi-objective optimization models and algorithms. These algorithms consider not only individual skills and motivation but also utilize Markov decision processes to incorporate probabilistic models of career development goals and aspirations. Bayesian networks are employed to model uncertainties in resource allocation decisions. The result is a strategic alignment of tasks with individual strengths. The optimization process is iterative, allowing organizations to refine resource allocation strategies dynamically.
1.5. Employee Benefits Optimization
Delving into the realm of employee benefits, OR brings a data-driven perspective to analyze diverse preferences and needs within the workforce. Indeed, it optimizes the design and allocation of employee benefits packages to meet the diverse needs of the workforce. It ensures that benefits are aligned with employee preferences while maximizing overall employee wellness. Indeed, it can help optimize the incentives and rewards for employees, such as bonuses, promotions, recognition, and feedback, to maximize their satisfaction and engagement.
Â
Employee Benefits Optimization considers not only traditional benefits but also lifestyle-related perks. This detailed optimization ensures that benefits packages are not only attractive but also genuinely contribute to the holistic well-being of employees. By tailoring benefits to individual preferences and needs, organizations can enhance employee satisfaction and create a positive workplace culture.
Â
From a technical point of view, Machine learning algorithms process data from various sources, including employee demographics and surveys, to identify trends and preferences. Decision trees and optimization algorithms are then utilized to tailor benefits packages, considering both traditional benefits and lifestyle-related perks. Continuous monitoring and analysis ensure benefits are not only attractive but also responsive to evolving employee needs, contributing to a culture of well-being.
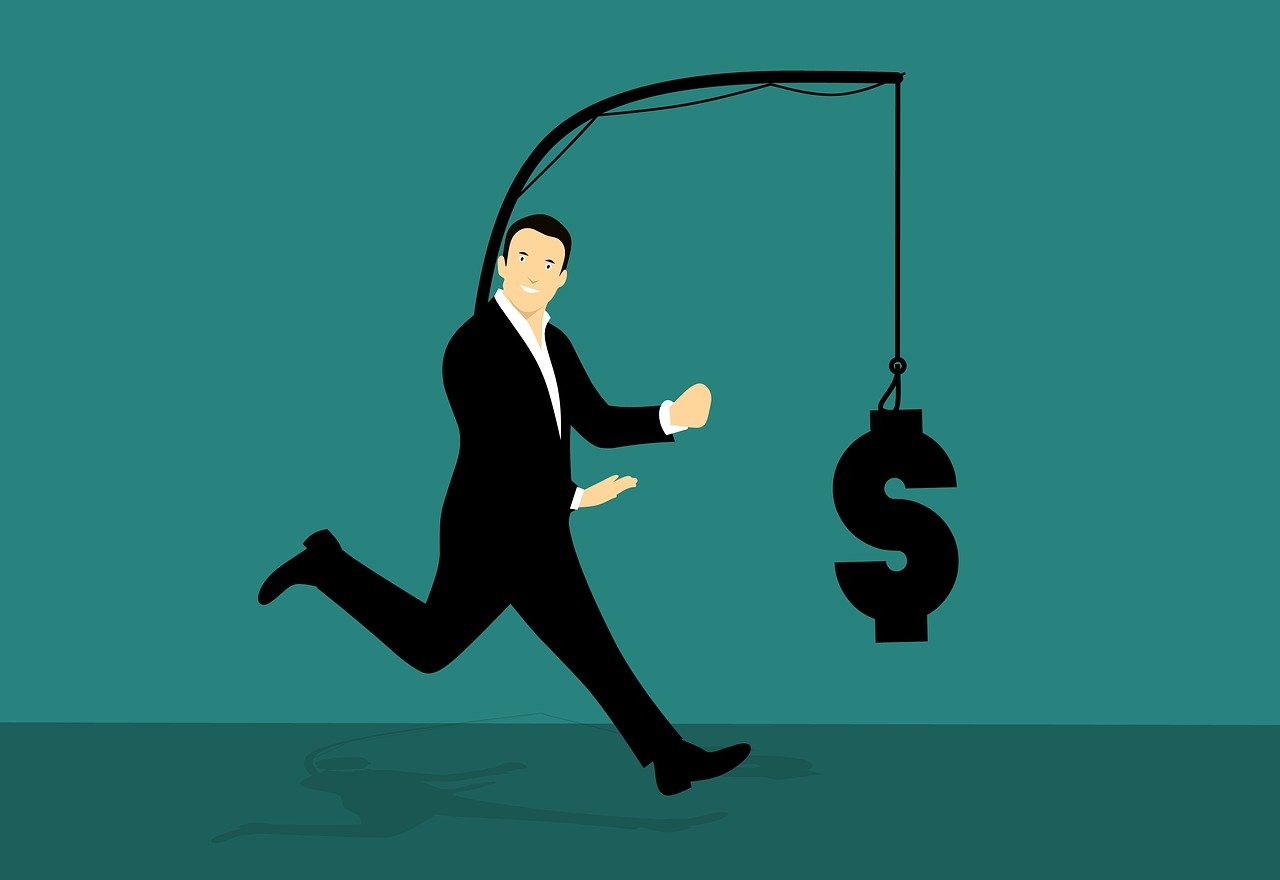
1.6. Cross-Training Optimization
Cross-training is a strategic investment in employee development, and OR optimizes training programs by conducting thorough skill assessments. It identifies skill gaps and tailors training content accordingly, ensuring efficiency in skill development. This data-driven approach ensures that cross-training is not only efficient but also aligned with the long-term career goals of employees. By investing in cross-training optimization, organizations can enhance employee skill sets, promote internal mobility, and cultivate a workforce that is adaptable and resilient. The technical sophistication in cross-training optimization ensures efficient skill development aligned with both immediate job requirements and long-term career goals.
1.7. Task Assignment Optimization
Task assignment, when optimized by OR algorithms, goes beyond mere skill matching. It delves into understanding employee strengths, weaknesses, and personal interests. By considering the psychological aspects of task assignments, OR ensures that employees are not just productive but also engaged and motivated. This approach significantly contributes to overall job satisfaction and organizational success. Through task assignment optimization, organizations can create a work environment where employees feel a sense of purpose and fulfillment in their roles.
Â
Task assignment optimization employs advanced optimization techniques, including integer programming and metaheuristic algorithms. Psychometric assessments are integrated into algorithms to understand employee strengths, weaknesses, and personality traits. Machine learning models analyze historical data to identify patterns of engagement and motivation, ensuring tasks are not only matched to skills but also to individual preferences. Dynamic programming is employed to adapt task assignments over time, considering changing employee dynamics and organizational goals. The result is a highly personalized and
adaptive approach to task assignments that contributes significantly to overall job satisfaction and organizational success.
2. Data Science Use Cases for Employee Well-Being
2.1. Health and Wellness Analytics
Data Science in health and wellness analytics involves a detailed exploration of health-related data, aiming to create an in-depth profile of each employee’s well-being. Beyond traditional metrics, this approach incorporates data from wearables and health assessments. By utilizing advanced analytical techniques, organizations can extract valuable insights that go beyond surface-level observations. These insights serve as the foundation for personalized health recommendations, fostering a workplace culture that prioritizes not only physical health but also mental well-being. This comprehensive approach to employee wellness contributes to a more holistic and proactive management of health within the workforce.
Â
In the realm of Health and Wellness Analytics, Data Science employs advanced statistical methods such as machine learning algorithms and predictive modeling. These techniques allow for the extraction of intricate patterns and trends from diverse health-related data sources, including wearables and health assessments. The application of natural language processing (NLP) in analyzing unstructured data, such as employee feedback and health reports, enhances the depth of insights. Additionally, the integration of Bayesian statistics enables the modeling of uncertainties, providing a more robust foundation for personalized health recommendations. Implementation may involve technologies like Apache Spark for large-scale data processing and Python-based frameworks such as TensorFlow or PyTorch for machine learning tasks.
2.2. Predictive Turnover Analytics
Predicting turnover goes beyond identifying potential departures; it involves a nuanced understanding of underlying factors. Data Science models in this context analyze a myriad of data points, ranging from traditional employee satisfaction surveys to project involvement metrics. This holistic approach allows organizations to take proactive measures, addressing potential issues before they escalate. By leveraging predictive analytics, organizations can significantly impact employee retention positively, creating a more stable and satisfied workforce. This data-driven strategy ensures that interventions are targeted and effective, ultimately enhancing the overall health of the organization.
Â
Predictive Turnover Analytics relies on sophisticated predictive modeling techniques, leveraging algorithms like Random Forests, Gradient Boosting, or Neural Networks. The analysis spans various data dimensions, including temporal patterns derived from project involvement timelines and sentiment analysis of employee communications. Advanced statistical methods like survival analysis may be employed to model time-to-event scenarios, predicting when an employee might consider leaving. Implementation may involve the use of data visualization tools like Tableau or Power BI for presenting actionable insights to stakeholders.
2.3. Employee Satisfaction Surveys
The traditional employee satisfaction survey undergoes a transformation with the integration of advanced analytics techniques. Sentiment analysis and predictive modeling add layers of depth to the insights derived from these surveys. The data science makeover enables organizations to uncover nuanced insights, guiding targeted interventions. This ensures that organizational improvements directly address the root causes of dissatisfaction, leading to a more content and engaged workforce. The marriage of traditional survey methods with data science enhances the effectiveness of organizational initiatives aimed at improving employee satisfaction.
Â
Predictive modeling, possibly using logistic regression or deep learning architectures, helps in identifying factors influencing satisfaction levels. The integration of tools like IBM Watson for sentiment analysis or
spaCy for text processing enhances the technical capabilities of the survey analysis. The results may be visualized using data visualization libraries like Matplotlib or Seaborn in Python.
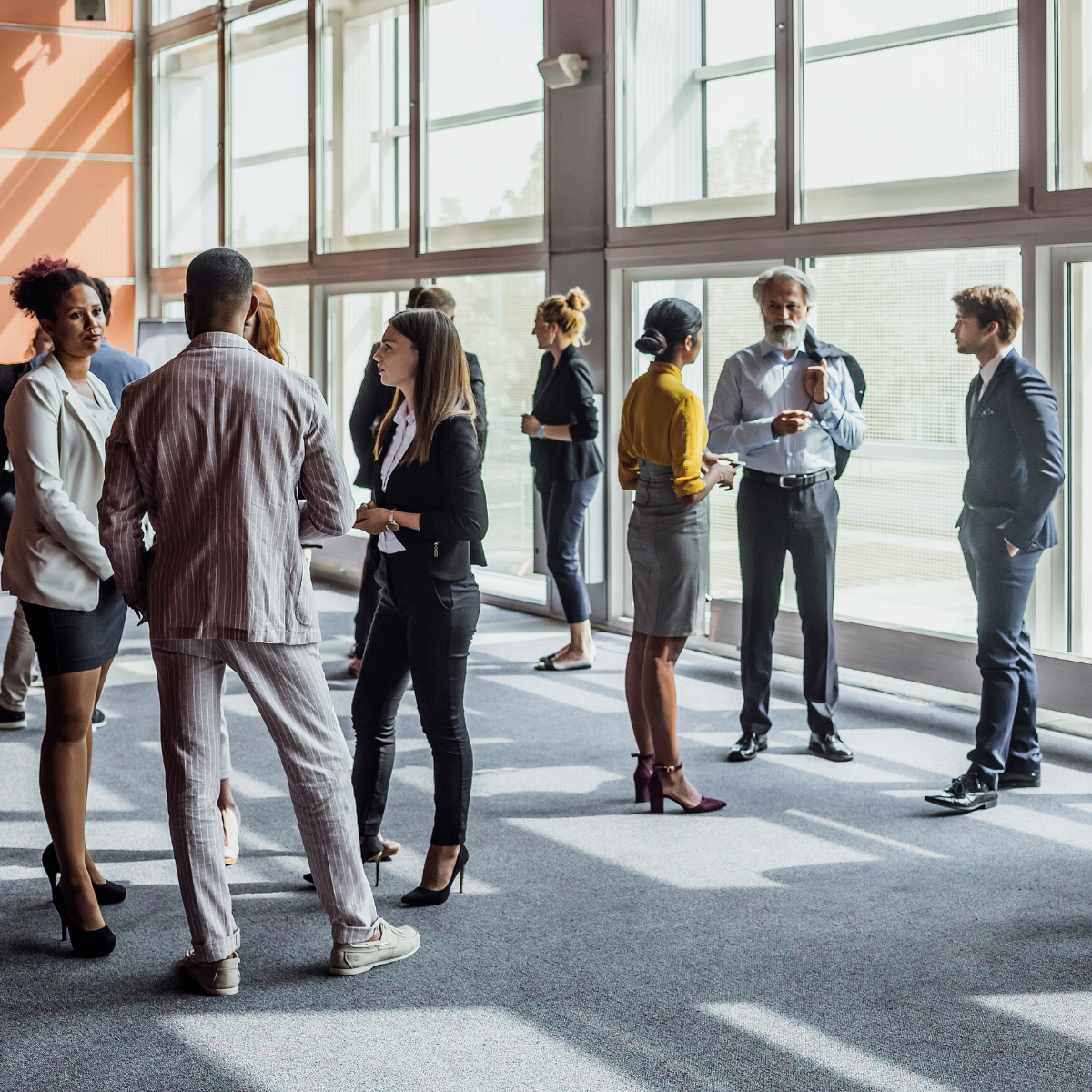
2.4. Diversity and Inclusion Analytics
Data Science plays a pivotal role in promoting diversity and inclusion within organizations. By analyzing metrics related to employee demographics, promotion rates, and workplace dynamics, organizations gain actionable insights. These insights guide strategies for fostering a more inclusive culture, contributing to the well-being of all employees. Data-driven diversity and inclusion initiatives not only address existing disparities but also create an environment where diverse perspectives are valued. This, in turn, enhances organizational health and creates a more vibrant and innovative workplace.
Â
Diversity and Inclusion Analytics utilize statistical methods such as regression analysis to identify correlations between employee demographics and workplace dynamics. Clustering algorithms, like k-means or hierarchical clustering, may be applied to group employees based on various attributes for a more granular analysis. Additionally, fairness-aware machine learning models are employed to ensure unbiased analysis and decision-making. The use of data anonymization techniques and differential privacy measures helps maintain data confidentiality while deriving actionable insights.
2.5. Employee Productivity Tracking
Data Science transforms the traditional understanding of productivity by incorporating qualitative data into the analysis. Collaboration patterns, task completion times, and even employee feedback become essential components of this holistic approach. By adopting a comprehensive view, organizations can
identify specific areas of improvement, leading to more meaningful enhancements in overall productivity and well-being. The integration of qualitative data provides a more accurate representation of employee contributions, allowing for targeted improvements that positively impact both individual and collective productivity.
Â
Employee Productivity Tracking incorporates advanced analytics techniques such as network analysis to understand collaboration patterns within teams. Time-series analysis may be employed to identify temporal patterns in task completion times, and sentiment analysis applied to employee feedback for a comprehensive view. Technical implementations may involve the use of version control systems like Git to track collaborative projects and the application of natural language processing libraries like NLTK or spaCy for sentiment analysis on textual data.
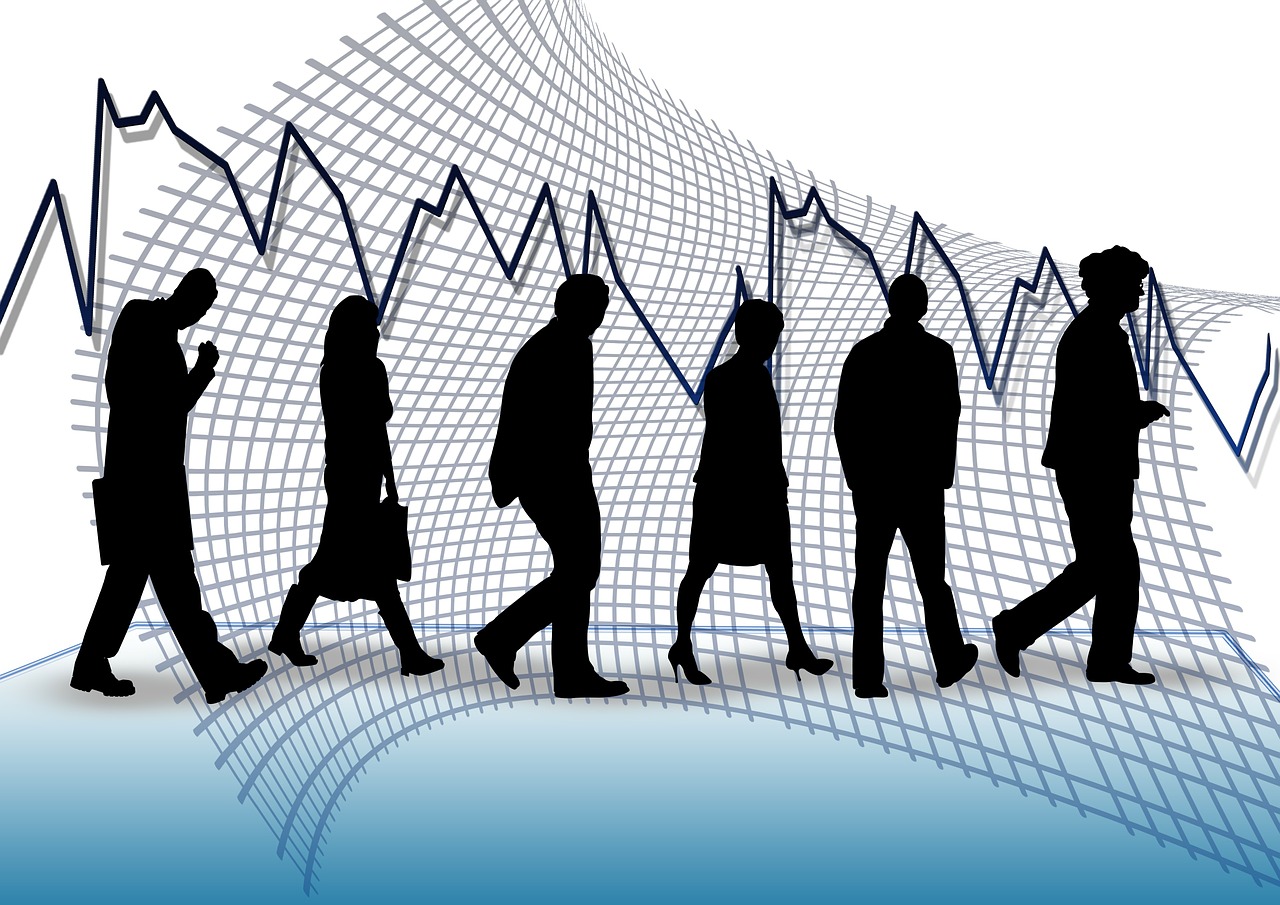
2.6. Employee Health Risk Assessment
In the realm of employee health, Data Science not only predicts potential health issues but also takes a proactive stance by providing tailored preventive measures. Through the analysis of historical health data, lifestyle choices, and environmental factors, organizations can develop personalized wellness programs. This proactive approach not only reduces health risks but also enhances overall employee well-being. The combination of predictive analytics and personalized interventions creates a workplace environment that actively supports and promotes the health of its employees, fostering a culture of well-being and preventive health management.
Â
Â
In the domain of Employee Health Risk Assessment, machine learning models, including logistic regression or decision trees, are utilized to predict health risks based on historical health data. Bayesian networks may be applied to model complex relationships between lifestyle choices and health outcomes.
Technologies like Apache Hadoop for distributed data processing and cloud-based platforms such as AWS Sagemaker for deploying machine learning models at scale contribute to the technical infrastructure. The implementation may also involve the integration of IoT devices for real-time health data collection, enhancing the accuracy of risk assessments.
3. Artificial Intelligence (AI) Use Cases for Employee Well-Being
3.1. Employee Engagement
AI-driven tools for gauging and improving employee engagement are built on sophisticated algorithms, utilizing advanced technologies such as natural language processing (NLP) and machine learning. These tools move beyond surface-level metrics and delve into a deep understanding of engagement dynamics. Sentiment analysis, combined with machine learning, enables the identification of intricate patterns in employee behavior and preferences. This nuanced understanding empowers organizations to implement highly targeted strategies, fostering a workplace environment that enhances overall engagement and job satisfaction.
Â
The algorithms employed in these tools utilize advanced statistical models and neural networks, allowing for real-time analysis of employee interactions and sentiments. Additionally, predictive analytics capabilities enable organizations to anticipate engagement trends and proactively address potential issues.
3.2. Mental Health Support Chatbots
AI-powered chatbots for mental health support represent a groundbreaking advancement in employee well-being initiatives. These chatbots are equipped with natural language processing and empathy algorithms, enabling them to offer immediate and personalized assistance. Beyond merely providing resources, these chatbots engage in meaningful conversations, tailoring their support to individual needs. This proactive approach to mental health contributes significantly to overall well-being, creating a supportive environment within the workplace.
Â
The natural language processing capabilities are enhanced by sentiment analysis algorithms, allowing the chatbots to detect subtle emotional cues in text. Empathy algorithms are based on extensive datasets of human emotions, enabling the chatbots to respond with genuine understanding and support.
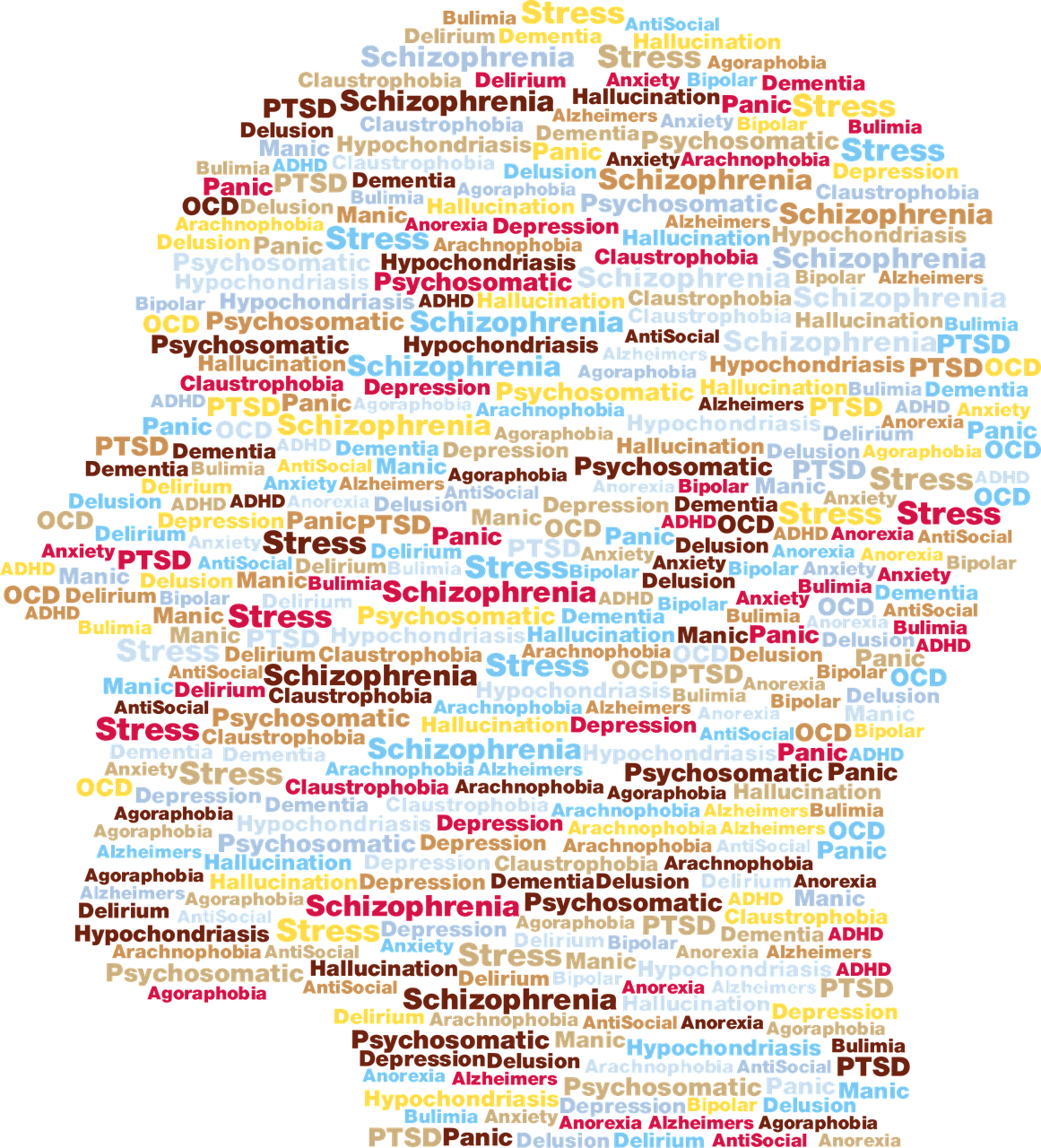
3.3. Automated Onboarding Processes
AI-driven automation revolutionizes the onboarding process, creating a seamless and personalized experience for new employees. From handling paperwork to organizing training schedules, every aspect of onboarding is streamlined through intelligent automation. Natural language processing enables chatbots to efficiently answer queries, ensuring that new employees feel supported and integrated from their very first day. This AI-driven onboarding approach contributes to a positive and stress-free entry into the organization, setting the tone for a productive and fulfilling work experience.
Â
Robotic Process Automation (RPA) is integrated into the onboarding processes, automating repetitive tasks and reducing manual intervention. Chatbots use advanced language models to understand and respond to queries with a high level of accuracy.
3.4. AI-driven Recognition Systems
Traditional recognition systems often lack personalization, but AI changes this by recognizing and understanding individual achievements at a granular level. Machine learning algorithms analyze performance data, going beyond quantitative metrics to identify qualitative contributions. This results in a recognition system that resonates with employees on a personal level, contributing to the cultivation of a positive and motivating work culture.
Â
The machine learning algorithms leverage clustering techniques to group similar achievements and contributions, ensuring a more nuanced and personalized recognition process. Predictive modeling anticipates upcoming achievements, allowing organizations to proactively acknowledge and celebrate employee successes.
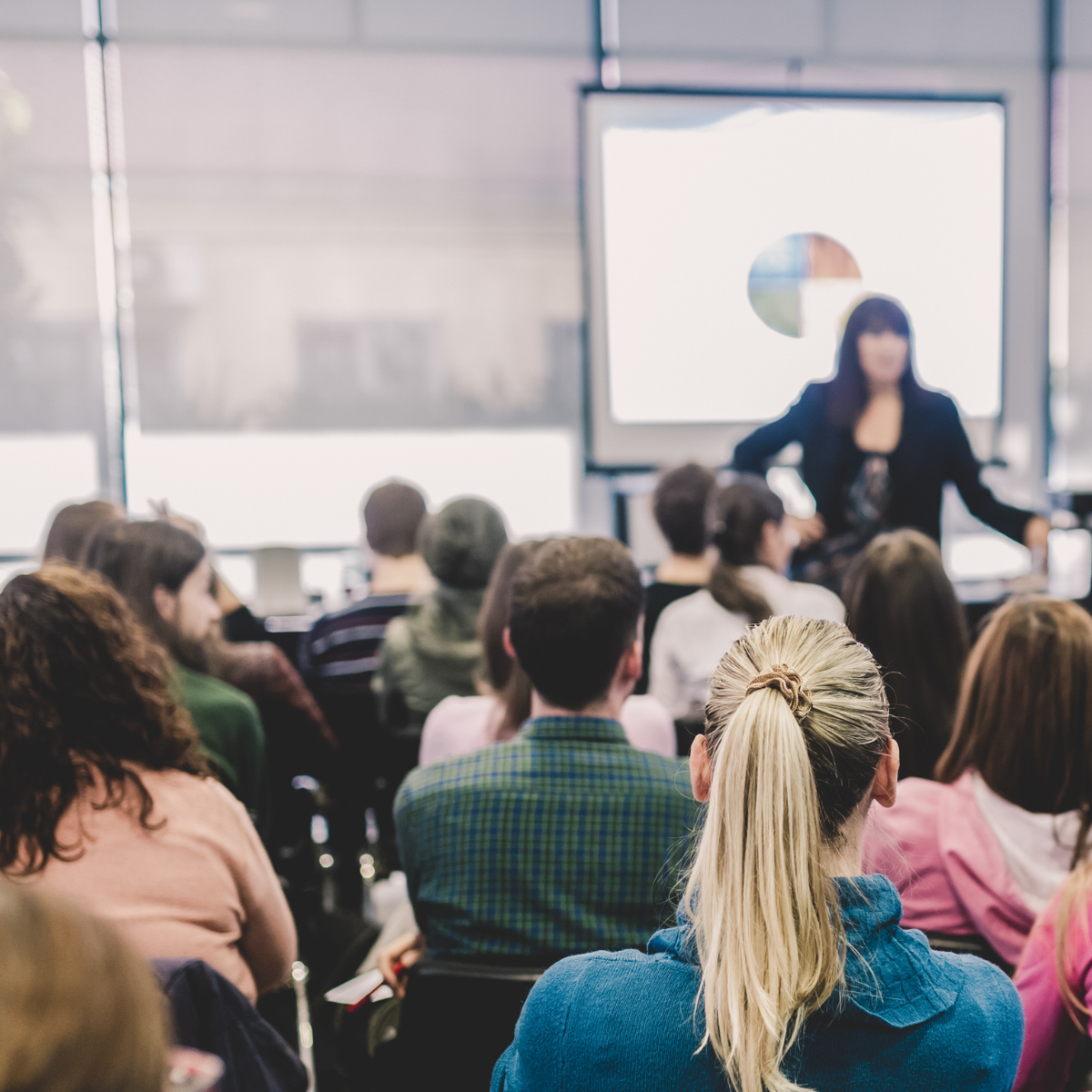
3.5. Personalized Learning and Development
AI transforms learning and development into a highly personalized journey, leveraging data analytics to analyze individual skills, career goals, and learning preferences. AI recommends tailored training programs, ensuring that employees receive targeted development opportunities. This not only enhances employee skills but also fosters a sense of individual growth and development, ultimately leading to heightened job satisfaction and overall well-being.
Â
The AI algorithms incorporate adaptive learning methodologies, adjusting training content based on individual progress and learning styles. Continuous feedback loops enable the system to dynamically adapt recommendations as employees acquire new skills and competencies.
Conclusion
In conclusion, the fusion of Operations Research, Data Science, and Artificial Intelligence heralds a new era in the quest for enhanced employee well-being. As organizations increasingly recognize the intrinsic link between a thriving workforce and operational success, the strategic application of these technologies becomes paramount. From optimizing schedules to predicting turnover and fostering mental health support, the synergistic use of OR, Data Science, and AI paints a comprehensive canvas of workplace transformation.
Â
The journey toward employee well-being is no longer confined to traditional methods; it is now a data-driven, algorithmically optimized endeavor. The positive impact of these technologies is not only seen in increased productivity but also in the creation of workplace cultures that prioritize the holistic development and satisfaction of every individual. As we look ahead, the continued integration of OR, Data Science, and AI promises to shape workplaces that not only adapt to change but proactively contribute to the well-being and success of the individuals who form the heart of every organization.