Data Strategy & Governance
We redefine what data can do for you with our data strategy & governance services.
A data strategy delineates an organization’s outlook on gathering, storing, disseminating, and utilizing its data. It simplifies the process of handling data at each stage of its journey, benefiting all individuals within the organization who require access to it.
The results of effectively implementing and regularly reassessing a Data Strategy contribute to transforming data into a valuable asset that consistently enhances organizational value. This strategic approach enables the organization to monetize data that has been strategically defined.
Data Strategy Workshop
Data Governance
Data governance is a systematic approach that guarantees data is in optimal condition to facilitate business initiatives and operations. Aligning data governance with business objectives offers numerous advantages.
The primary challenge in data governance strategy lies in aligning it with business initiatives rather than just advocating its value independently. This entails ensuring that data governance actively supports core business objectives like enhancing data accessibility and addressing data quality issues.
Data Services Offered
Data Strategy Exec Workshop
Software & Platform Eval Sessions
Architecture Design Session
ETL Services
Data Warehouse Health Checks
Data Migrations Services
Data Integration Services
Data Quality & Catalog Services
Technologies We Support
Enroll for
Use Cases
Dive into the Data use cases by Industry to learn about industry-specific solutions. Our Industries Page is a gateway to discovering how we impact businesses across various sectors.
Success Stores
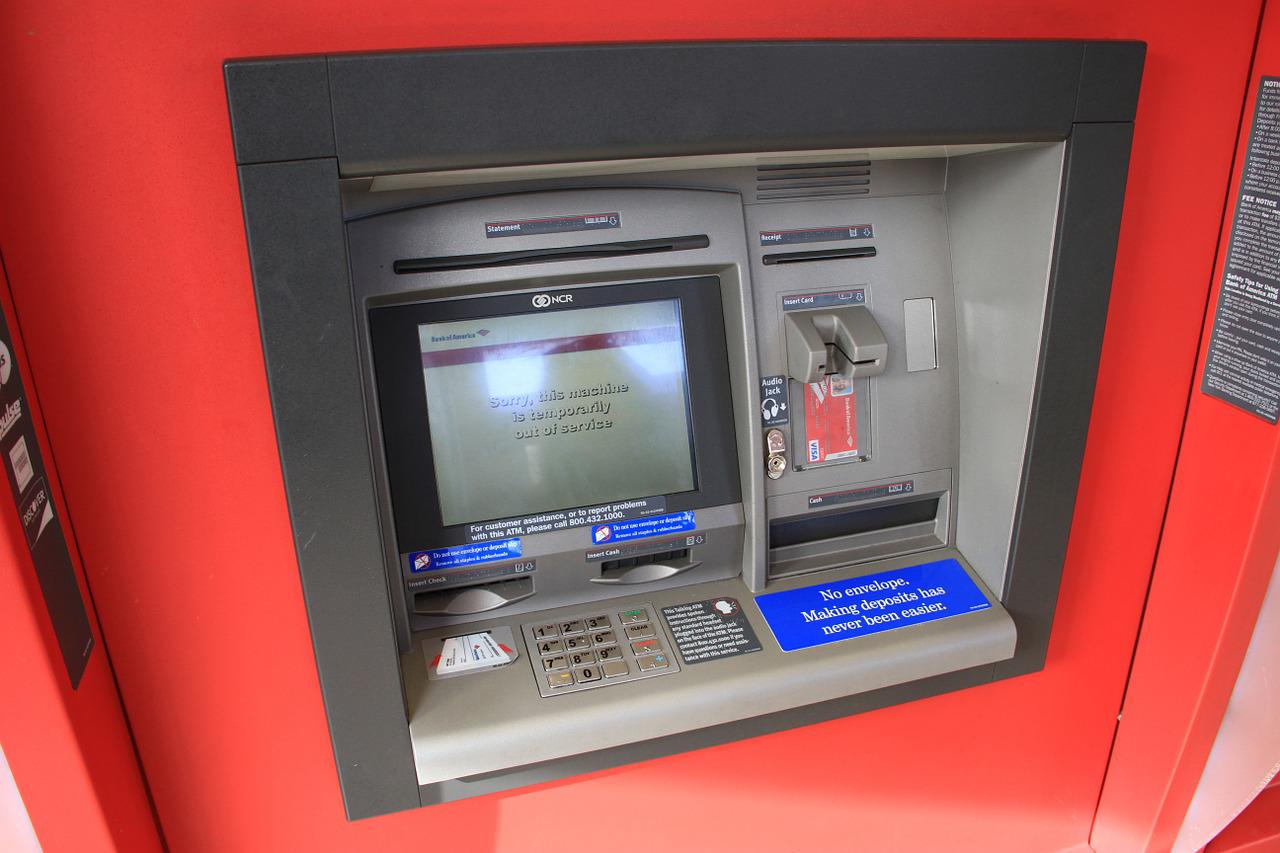
Yapı Kredi Bank
Yapı Kredi had launched a data governance initiative and needed to create a business glossary as part of that effort. The bank used the IBM® InfoSphere® Information Governance Catalog offering to create and define common business terms and to foster greater collaboration among its data stewards and data managers.
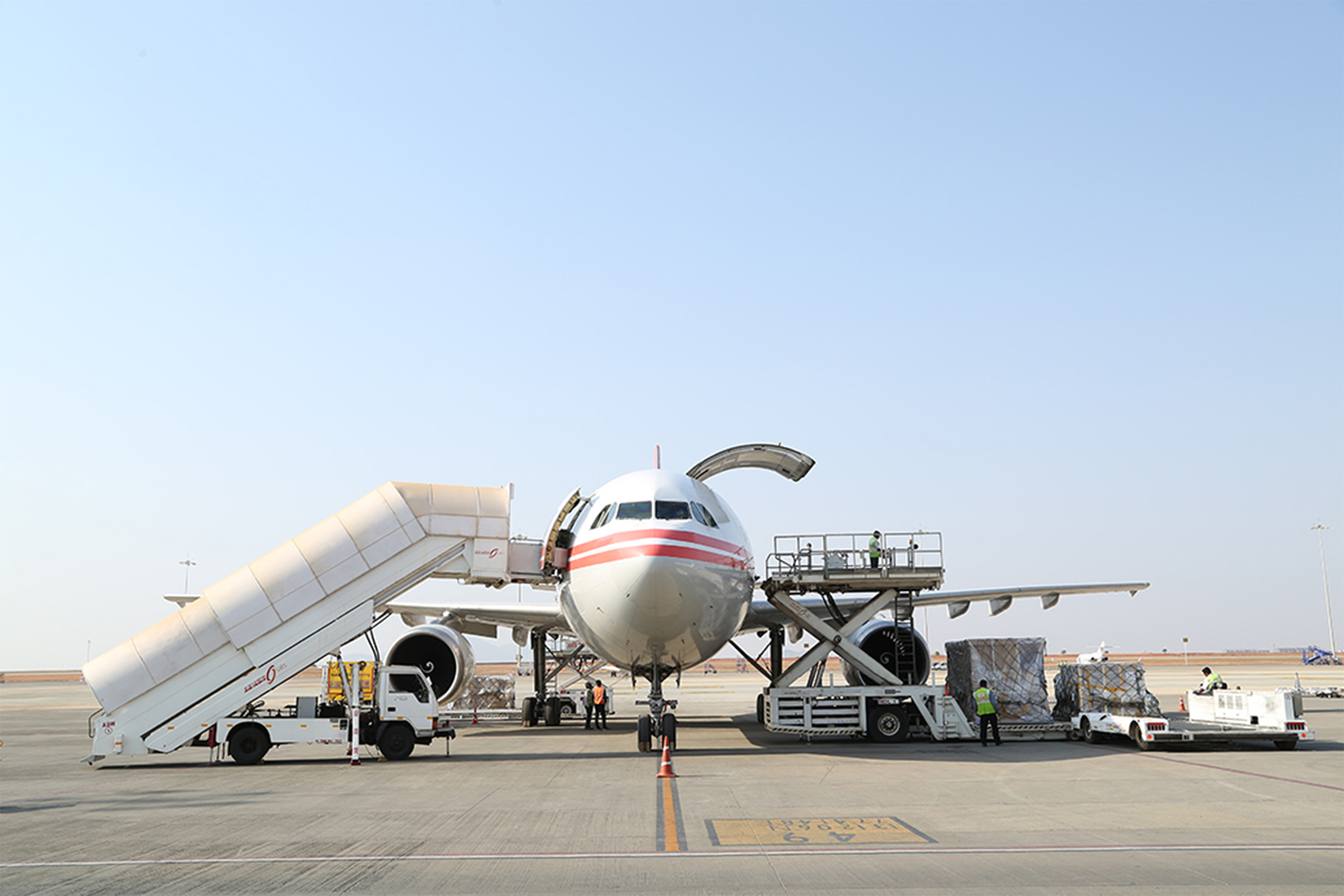
Air India SATS Airport Services Private Limited (AISATS)
When Air India SATS Airport Services Private Limited (AISATS) needed to migrate its SAP Business One platform from an on-premises data center to the cloud, it chose IBM Cloud® as its provider. The teamwork between IBM and AISATS was outstanding, and the new implementation has generated significant cost savings and productivity gains.
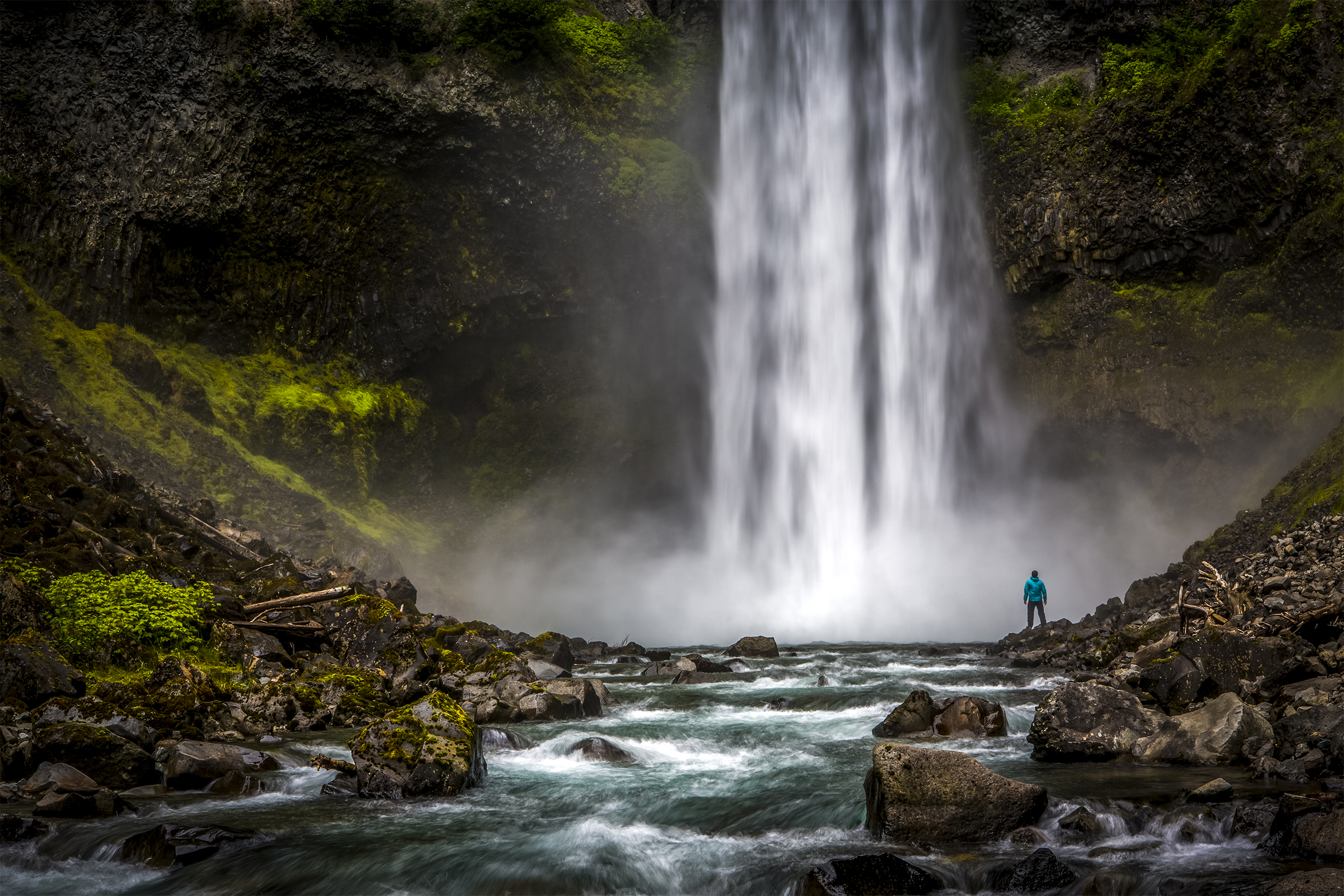
Celestica
Celestica uses the IBM Envizi Sustainability Performance Management suite to help consolidate and navigate ESG data and streamline reporting.
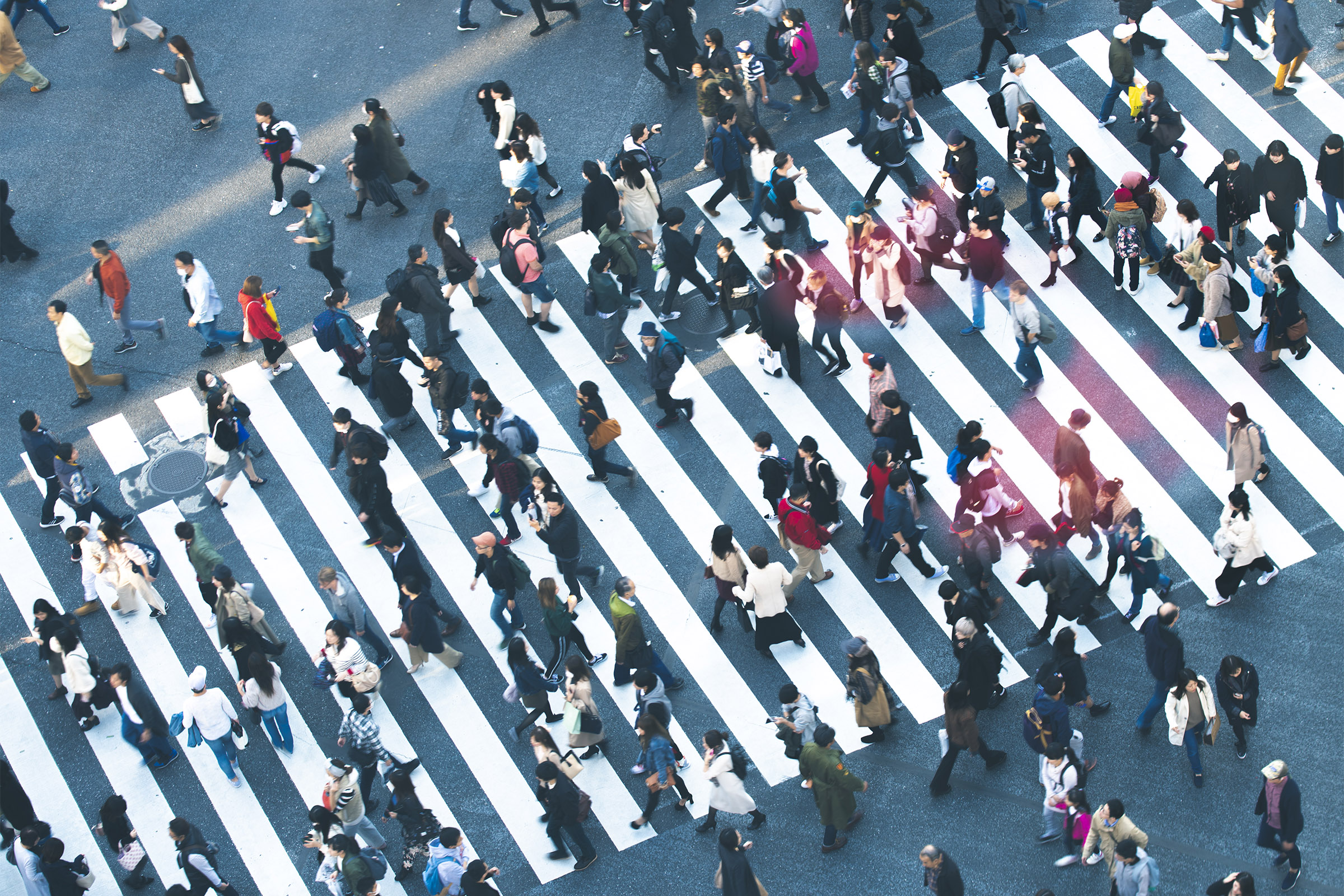
University of Fukui Hospital
University of Fukui Hospital adopted a hybrid cloud strategy to build a highly secure and highly usable platform as a system platform for handling medical data.
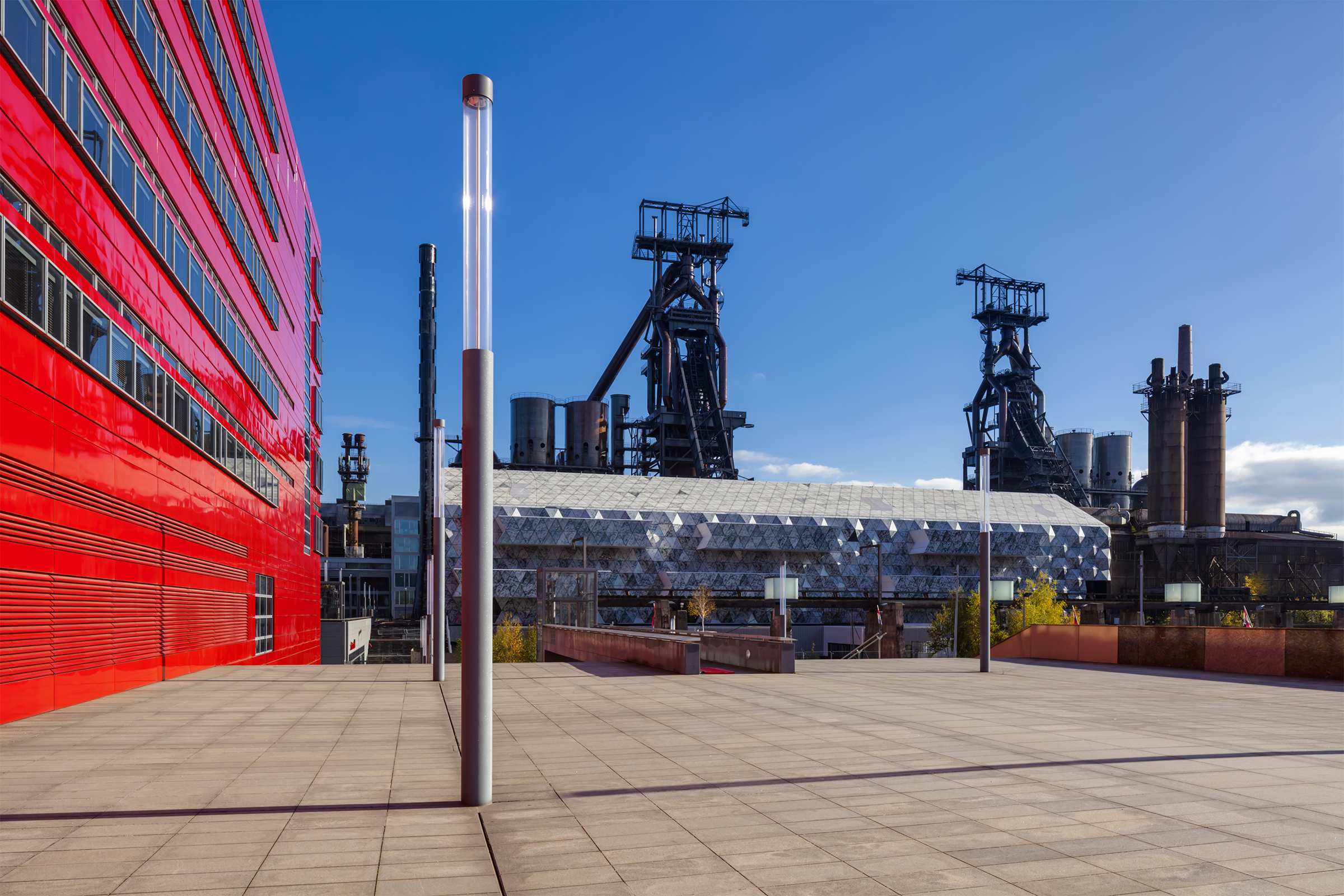
Luxembourg Institute of Science and Technology
Working with IBM, LIST built a unique data analytics and visualization platform designed to empower companies and researchers in Luxembourg to test AI projects.
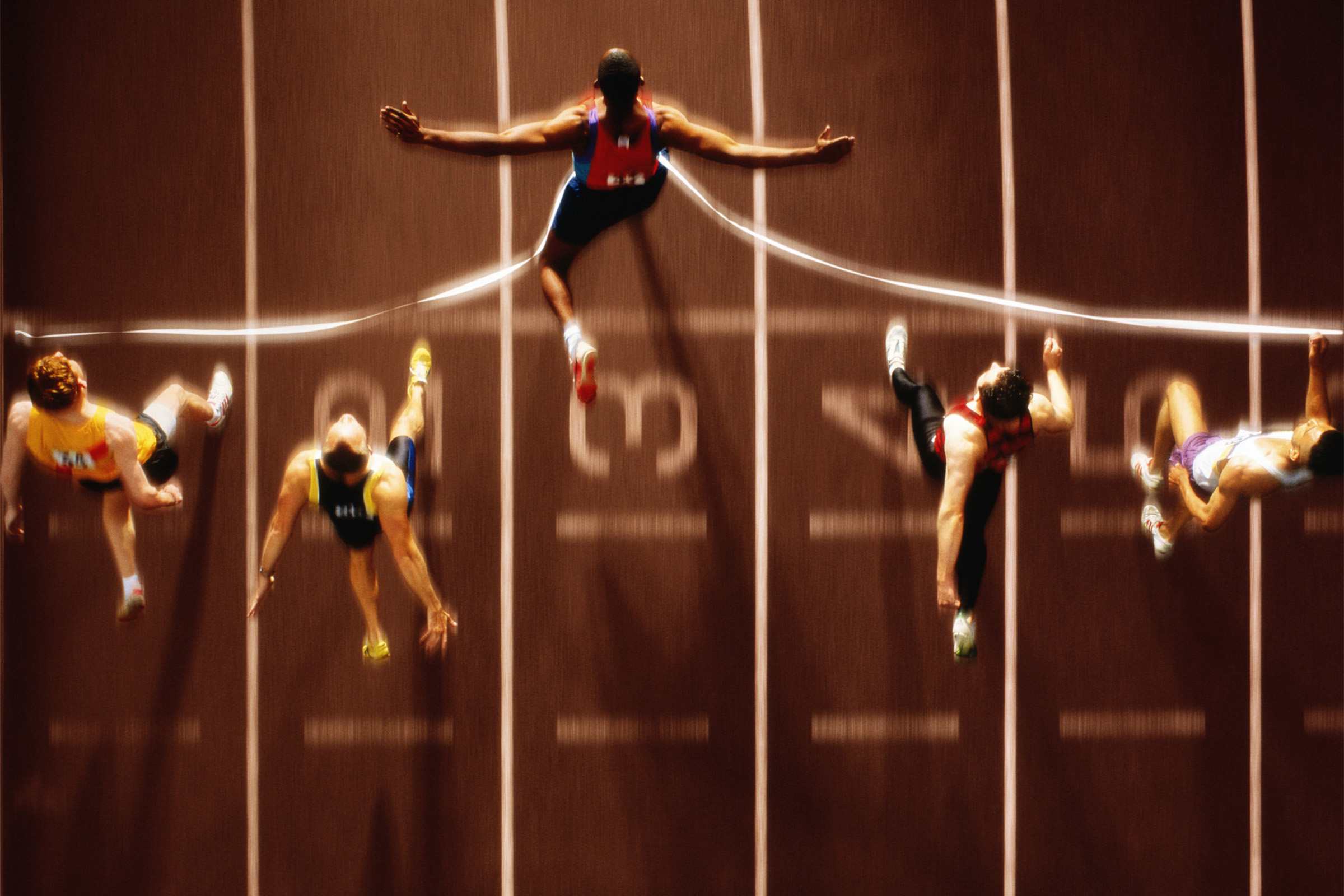
Puma
To enable its production database to scale to power microservices with 24x7 availability, PUMA deployed the IBM Db2 pureScale Feature in two server clusters. Now, the database supports 300% –400% more users and can be maintained without taking it offline.
Resources
Data Security
Prevent Fraud, Waste, and Abuse: Secure Your Company’s Reputation with Cresco’s Advanced Security Analytics Solutions.
Contact for Product Demo
We provide tailor-made software demos. Contact us today to schedule a demo that fits your needs.
Tech Consulting
We provide support in tailor-made software development, seamless systems integration, or adept IT project management.
Visit Cresco's
Featured Blogs
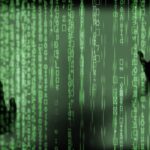
Data Strategy & Governence Challenges
FAQs
A data strategy is a comprehensive plan that outlines how an organization will leverage data to achieve its business objectives. It encompasses the collection, storage, analysis, and usage of data to inform decision-making and drive innovation.
A data strategy is important because it provides a roadmap for organizations to effectively manage and utilize their data assets. It ensures that data is aligned with business goals, promotes data-driven decision-making, enhances operational efficiency, and enables innovation and competitive advantage.
Key components of a data strategy typically include:
- Vision and objectives: Clear articulation of the organization’s goals and objectives related to data.
- Governance framework: Policies, processes, and roles for managing data effectively, including data privacy and security measures.
- Data architecture: Design and structure of data storage, integration, and analytics systems.
- Data quality management: Procedures for ensuring data accuracy, completeness, and consistency.
- Data lifecycle management: Strategies for managing data from acquisition to disposal.
- Data analytics and insights: Approaches for extracting valuable insights from data to support decision-making.
- Data culture: Fostering a culture that values data-driven decision-making and encourages data literacy among employees.
Developing a data strategy involves several steps:
- Understand business objectives: Align the data strategy with the organization’s overall goals and objectives.
- Assess current state: Evaluate existing data infrastructure, processes, and capabilities.
- Identify gaps and opportunities: Determine areas where improvements or new initiatives are needed to support business objectives.
- Define the strategy: Develop a comprehensive plan that outlines the vision, goals, and key components of the data strategy.
- Implement and monitor: Execute the strategy, monitor progress, and make adjustments as needed to ensure alignment with business needs.
Successful implementation of a data strategy requires:
- Leadership support: Strong commitment from executive leadership to prioritize and champion data initiatives.
- Cross-functional collaboration: Collaboration across departments to ensure alignment of data initiatives with business objectives.
- Investment in technology and resources: Adequate investment in data infrastructure, tools, and talent to support the execution of the strategy.
- Change management: Effective communication and training to foster a data-driven culture and facilitate adoption of new processes and technologies.
- Continuous improvement: Regular review and adjustment of the data strategy based on feedback and changing business needs.
Data governance refers to the framework, policies, processes, and procedures that organizations put in place to ensure that their data is managed effectively, securely, and in compliance with regulations and internal policies. It involves defining roles and responsibilities, establishing standards for data quality and integrity, and implementing controls to protect sensitive information.
Data governance is important because it helps organizations maximize the value of their data assets while minimizing risks associated with data misuse, breaches, or inaccuracies. It promotes data quality, consistency, and reliability, which are essential for informed decision-making, regulatory compliance, and maintaining trust with stakeholders.
Key components of data governance typically include:
- Data policies and standards: Documented guidelines and rules governing how data is collected, stored, processed, and accessed.
- Data stewardship: Designation of individuals or teams responsible for overseeing and managing specific data assets or domains.
- Data quality management: Processes and procedures for ensuring that data is accurate, complete, and consistent.
- Data security and privacy: Measures to protect data from unauthorized access, breaches, or misuse, and ensure compliance with relevant regulations.
- Metadata management: Management of metadata, including data definitions, lineage, and usage, to facilitate data discovery and understanding.
- Data lifecycle management: Policies and procedures for managing data throughout its lifecycle, from creation to archival or deletion.
- Data compliance and risk management: Processes for ensuring compliance with data-related regulations and managing risks associated with data governance.
Establishing a data governance framework involves several steps:
- Define objectives: Clearly articulate the goals and objectives of the data governance initiative, aligning them with the organization’s overall strategy and priorities.
- Assess current state: Evaluate existing data governance practices, processes, and capabilities to identify strengths, weaknesses, and areas for improvement.
- Develop governance structure: Define roles, responsibilities, and organizational structures for governing data, including the establishment of a data governance council or steering committee.
- Establish policies and standards: Develop documented policies, standards, and guidelines governing data management, quality, security, privacy, and compliance.
- Implement processes and controls: Define processes and procedures for managing data governance activities, including data stewardship, quality assurance, risk management, and compliance monitoring.
- Provide training and communication: Educate stakeholders about the importance of data governance, their roles and responsibilities, and the policies and procedures they need to follow.
- Monitor and review: Continuously monitor and evaluate the effectiveness of the data governance framework, making adjustments as needed to address emerging issues or changing business requirements.
Successful implementation of data governance requires:
- Executive sponsorship: Strong support and involvement from executive leadership to prioritize and champion data governance initiatives.
- Cross-functional collaboration: Collaboration across departments and stakeholders to ensure alignment of data governance activities with business objectives and priorities.
- Clear communication: Transparent communication about the goals, benefits, and requirements of data governance, as well as the roles and responsibilities of stakeholders.
- Continuous improvement: Regular review and refinement of the data governance framework based on feedback, lessons learned, and changes in business needs or regulatory requirements.
- Technology enablement: Leveraging data governance tools and technologies to automate processes, enforce policies, and enable greater visibility and control over data assets.
- Data culture: Fostering a culture that values data governance and encourages accountability, ownership, and collaboration among stakeholders.
Metadata is data that provides information about other data. It describes various aspects of data, such as its structure, content, format, and context. Metadata helps users understand and manage data more effectively by providing essential information about its origins, usage, and characteristics.
Metadata is important because it enhances the usability, discoverability, and management of data. It provides valuable context and insights that help users understand data, assess its quality, and determine its relevance for specific purposes. Metadata also facilitates data integration, search, and retrieval, enabling more efficient data analysis and decision-making.
There are several types of metadata, including:
- Descriptive metadata: Provides information about the content, context, and structure of data, such as titles, descriptions, keywords, and tags.
- Structural metadata: Describes the organization and relationships of data elements within a dataset, such as data schemas, tables, fields, and data models.
- Administrative metadata: Includes information about the management and administration of data, such as ownership, access rights, version history, and data provenance.
- Technical metadata: Describes the technical characteristics and specifications of data, such as file formats, data types, data sizes, and data encoding.
- Use metadata: Records information about the usage and access patterns of data, such as access logs, usage statistics, and user annotations.
Metadata is used in various aspects of data management, including:
- Data discovery: Metadata helps users find and identify relevant data assets by providing descriptive information and search capabilities.
- Data integration: Metadata facilitates the integration of disparate data sources by describing their structure, format, and relationships.
- Data quality: Metadata supports data quality management efforts by providing information about data lineage, accuracy, completeness, and consistency.
- Data governance: Metadata enables the enforcement of data governance policies and controls by documenting ownership, access rights, and compliance requirements.
- Data lineage: Metadata tracks the lineage of data from its source to its destination, helping users understand data transformations, dependencies, and impacts.
- Data security: Metadata assists in managing data security and privacy by documenting access controls, encryption, and masking requirements.
Managing metadata effectively involves several steps:
- Identify metadata requirements: Determine the types of metadata needed to support specific business objectives, processes, and use cases.
- Define metadata standards: Establish standards and guidelines for metadata creation, documentation, and maintenance to ensure consistency and quality.
- Capture metadata: Collect metadata from various sources and systems using automated tools, manual entry, or extraction from data sources.
- Store and organize metadata: Store metadata in a centralized repository or metadata catalog, organized in a structured manner for easy access and retrieval.
- Maintain metadata: Regularly update and maintain metadata to keep it accurate, relevant, and up-to-date, reflecting changes in data assets and business requirements.
- Govern metadata: Implement metadata governance processes to ensure compliance with metadata standards, policies, and regulations, and to manage metadata lifecycle.
- Integrate metadata management: Integrate metadata management capabilities into data management processes, tools, and systems to enable seamless metadata exchange and interoperability.
Some common challenges in metadata management include:
- Lack of metadata standards: Absence of standardized metadata formats and definitions can lead to inconsistency and interoperability issues.
- Data silos: Fragmentation of metadata across disparate systems and applications makes it difficult to discover and manage metadata effectively.
- Metadata quality: Poor quality metadata, such as incomplete, inaccurate, or outdated information, can undermine the usability and reliability of metadata.
- Metadata governance: Inadequate governance processes and controls can result in inconsistent metadata management practices and compliance issues.
- Data lineage complexity: Complex data environments and data transformations can make it challenging to track and document data lineage accurately.
- Resource constraints: Limited resources, including budget, expertise, and tools, may hinder organizations’ ability to invest in metadata management initiatives.
- Cultural barriers: Resistance to change, lack of awareness, and organizational silos can impede collaboration and communication around metadata management efforts.